Automate Your Data Warehouse
Deliver robust analytics faster with automation: slash manual coding, ensure scalable and trustable data warehousing.
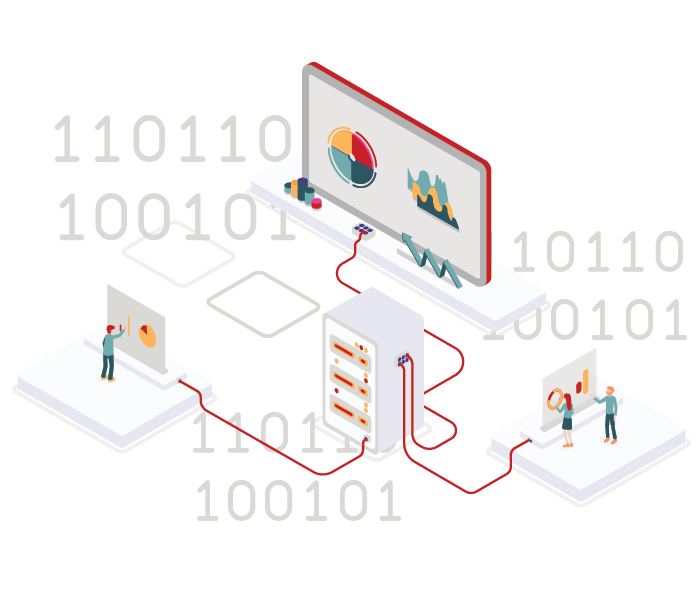
Build Data Warehouses Faster
Faster, Simpler Delivery
Automate up to 95% of coding to accelerate data warehouse projects. Reduce errors, speed-up development and launch at scale, all within days.
- Automated code generation.
- Up to 95% less manual coding.
- Days-to-weeks deployment.
Consistency & Standards
Ensure every workflow adheres to proven coding standards with built-in best practices. Streamline maintenance – despite staff turnover or new data.
- Built-in best practices.
- Survive staff turnover.
- Seamless new data sources.
Reliable Analytics
Centralize and govern data for trustworthy insights. Confidently power reports and dashboards – future-proof your analytics strategy, at scale.
- Trusted, governed pipelines.
- Transparent data lineage.
- Consistent reporting logic.
The Automation Engine
See how WhereScape streamlines each stage, from discovery to deployment. Our metadata-driven approach reduces complexity, errors and increases time-to-value.
Discover
Model
Develop
Deploy
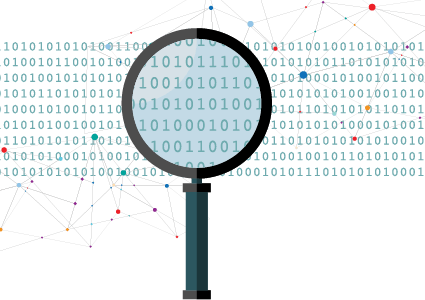
Profile Sources and Identify Key Requirements
WhereScape automates source profiling to reveal relationships, data quality, and conflicts. By understanding your data upfront, you reduce reworking and align business requirements from the beginning.
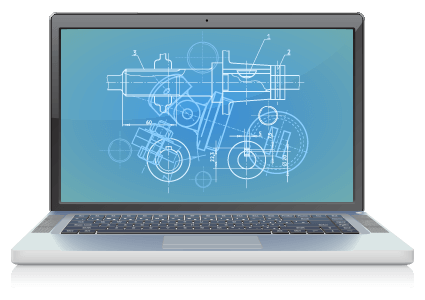
Build Conceptual and Physical Data Models
Leverage drag-and-drop modeling, built-in best practices, and validations to shape your ideal architecture. Instantly convert conceptual designs into physical models for consistent, error-free structures.
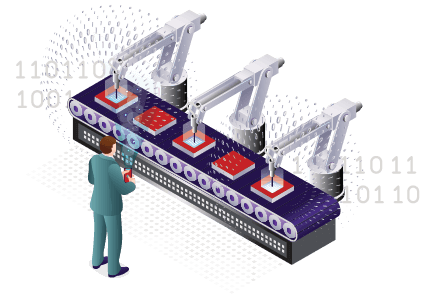
Auto-Generate Code and Validate Data Flows
WhereScape automatically generates native SQL or platform-specific code, removing the need for manual scripting. Validate transformations in minutes, catching issues before they become bigger problems.
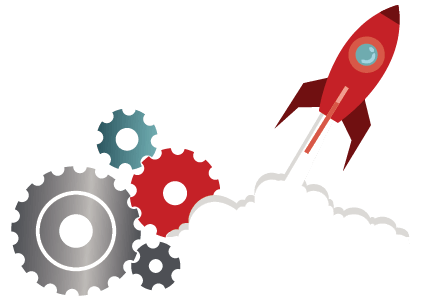
Automate Release and Scale Data Infrastructure
Accelerate deployment with integrated orchestration and scheduling. Track changes via version control and easily adapt to new demands without sacrificing performance or stability.
Recommended Resources
Dive deeper with our collection of linked resources on data warehouse automation. Gain actionable guidance on how to automate your data initiatives.
Start Automating Now
Request your demo to see WhereScape in action and unlock the power of automation.