Join our fast-and-friendly virtual tour to...
Common Data Quality Challenges and How to Overcome Them
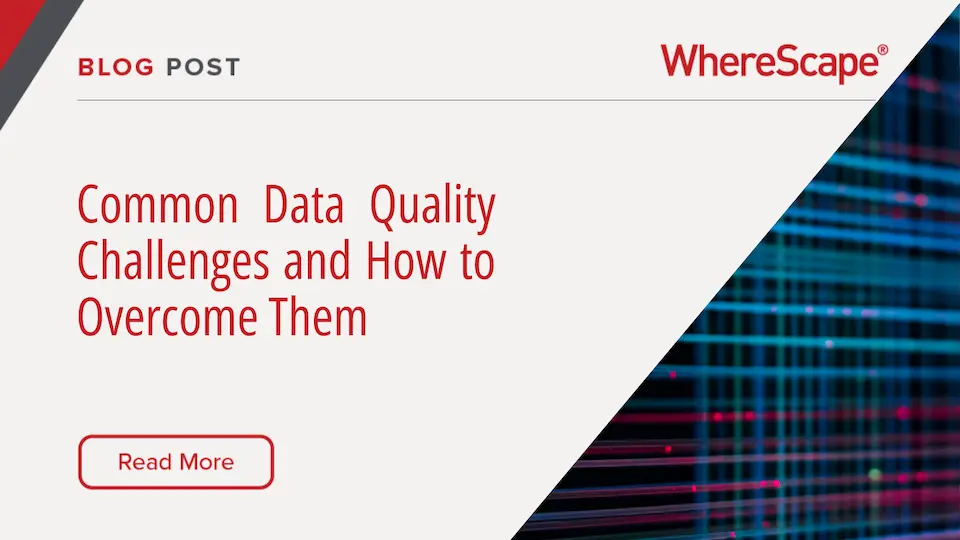
The Importance of Maintaining Data Quality
Improving data quality is a top priority for many forward-thinking organizations, and for good reason. Any company making decisions based on data should also invest time and resources into ensuring high data quality. Data quality refers to how accurate, consistent, and relevant a dataset is. Ensuring data integrity over time poses various data quality challenges, but there are tried and tested methods for overcoming them.
Data Quality Challenges in Modern Businesses
The countless facets of data quality and data management lead to intricate challenges that can be difficult to overcome. With a clear understanding of exactly what types of challenges impede your path to improving data quality and how to identify and overcome them, you can foster a healthier data environment for your company’s future. Most common data quality challenges stem from these issues:
- Inconsistent formatting
- Duplicate data
- Outdated data
- Data silos
- Poor data entry practices
- Unclear data standards
- Lack of data training
- Data security, access, and privacy problems
- Rapid growth
- Integration snags
- Lack of sufficient data governance and automation tools
Strategies for Improving Data Quality
In learning how to improve data quality, every organization must begin with their goals and key data uses in mind. Your unique data quality standards depend on the types of data you use to drive key decisions. With those priorities in mind, organizations can focus on key frameworks for maintaining and improving data quality.
Improving data quality involves setting clear data standards, implementing robust data validation processes, and regularly auditing data for errors. Training employees on data management best practices also ensures consistency and accuracy across the organization. Leveraging data governance frameworks helps maintain oversight and accountability for data quality initiatives.
How to Maintain Data Quality in Your Organization
The first step in how to maintain data quality is setting clear, measurable standards for all sources of data. Once rigorous standards are in place, implement validation processes and schedule frequent audits to catch errors early on. Tools like WhereScape Data Vault Express can help streamline these efforts
In order to keep up with the ongoing cleaning and validation required for high data quality, prioritize creating a culture of data ownership and accountability. When everyone who interacts with data in any capacity can recognize and understand their role in protecting data quality, your ability to maintain high data quality will benefit.
How to Improve Data Quality with Technology
Once you’ve built a foundation of ownership and routine cleaning to address data quality challenges, it’s time to determine which tools and technology can help you make further improvements. Even with a strong culture of data accountability, human error happens. Constant validation and cleaning can also eat away at skilled team member hours without the right tools.
In discovering how to improve data quality, Explore solutions like WhereScape’s automation software for automated data warehouses. With the help of advanced technology, such as WhereScape RED, you can drastically reduce the amount of time it takes to turn your raw data into actionable, reliable insights to drive decisions
Ensuring Data Integrity Across Your Organization
Ensuring data integrity involves protecting data throughout its lifecycle. Data security and automated tools are key to ensuring data integrity since some of the most common data quality challenges and risks to data integrity involve human error, data breaches, and system failures.
Implementing strong access controls, encryption, and documentation helps safeguard data integrity. Regularly updating security protocols and conducting integrity checks are essential to maintaining reliable data.
Best Practices for Ensuring Data Integrity
Ensuring data integrity requires strategic use of automation technology and strict protocols for data entry and security. Data automation tools help ensure data integrity by providing automated documentation and reducing human error from manual entry. They also provide scalable solutions for growing businesses instead of allowing silos to form or data loss to occur.
Additional steps for ensuring data integrity include conducting regular data security training, using reliable backup systems, and enforcing strict validation rules. Over time, especially during periods of growth, conduct audits to ensure your processes are still serving their intended purpose.
Continuous Improvement in Data Quality
Learning how to improve data quality is a journey, not a destination. While organizations can strive to meet data quality standards, perfection is always just out of reach and there is always more you can do to work toward a goal of improving data quality.
Continuous improvement is a guiding principle in this space, reminding data teams that growth over time is a worthy goal. Working toward that goal requires constantly reviewing and refining data processes and using feedback loops to determine areas for improvement. With a growth mindset, a culture of innovation, and an ever-advancing suite of data management tools, organizations can benefit from continuous improvement in data quality.
Overcoming Data Quality Challenges for Long-Term Success
The long-term success of your organization relies on gradual improvements to data processes. As you overcome data quality challenges, you open the door to data-driven decisions that can change the trajectory of your business.
Overcoming data quality challenges involves addressing root causes like outdated processes and implementing lasting solutions like automated data warehouses. With WhereScape, organizations make faster, more confident decisions based on reliable and accurate data. Book a demo to see how WhereScape can help you overcome data quality challenges.
WhereScape at TDWI Munich: Automate Data Vault on Databricks
WhereScape at TDWI Munich 2025: Automate a Full Data Vault on Databricks in Just 45 Minutes June 24–26, 2025 | MOC Munich, Germany As data complexity grows and business demands accelerate, scalable and governed data architectures are no longer optional—they're...
What Is OLAP? Online Analytical Processing for Fast, Multidimensional Analysis
Streamline your data analysis process with OLAP for better business intelligence. Explore the advantages of Online Analytical Processing (OLAP) now! Do you find it challenging to analyze large volumes of data swiftly? A Forrester study reveals that data teams spend...
Build AI-Ready Data: Visit WhereScape at AI & Big Data Expo
June 4–5, 2025 | Booth 202 | Santa Clara Convention Center As organizations scale their artificial intelligence and analytics capabilities, the demand for timely, accurate, governed, and AI-ready data has become a strategic priority. According to Gartner, through...
Automating Star Schemas in Microsoft Fabric: A Webinar Recap
From Data Discovery to Deployment—All in One Workflow According to Gartner, data professionals dedicate more than half of their time, 56%, to operational tasks, leaving only 22% for strategic work that drives innovation. This imbalance is especially apparent when...
What is a Data Model? How Structured Data Drives AI Success
What is a data model? According to the 2020 State of Data Science report by Anaconda, data scientists spend about 45% of their time on data preparation tasks, including cleaning and loading data. Without well-structured data, even the most advanced AI systems can...
ETL vs ELT: What are the Differences?
In working with hundreds of data teams through WhereScape’s automation platform, we’ve seen this debate evolve as businesses modernize their infrastructure. Each method, ETL vs ELT, offers a unique pathway for transferring raw data into a warehouse, where it can be...
Dimensional Modeling for Machine Learning
Kimball’s dimensional modeling continues to play a critical role in machine learning and data science outcomes, as outlined in the Kimball Group’s 10 Essential Rules of Dimensional Modeling, a framework still widely applied in modern data workflows. In a recent...
Automating Data Vault in Databricks | WhereScape Recap
Automating Data Vault in Databricks can reduce time-to-value by up to 70%—and that’s why we hosted a recent WhereScape webinar to show exactly how. At WhereScape, modern data teams shouldn't have to choose between agility and governance. That's why we hosted a live...
WhereScape Recap: Highlights From Big Data & AI World London 2025
Big Data & AI World London 2025 brought together thousands of data and AI professionals at ExCeL London—and WhereScape was right in the middle of the action. With automation taking center stage across the industry, it was no surprise that our booth and sessions...
Why WhereScape is the Leading Solution for Healthcare Data Automation
Optimizing Healthcare Data Management with Automation Healthcare organizations manage vast amounts of medical data across EHR systems, billing platforms, clinical research, and operational analytics. However, healthcare data integration remains a challenge due to...
Related Content
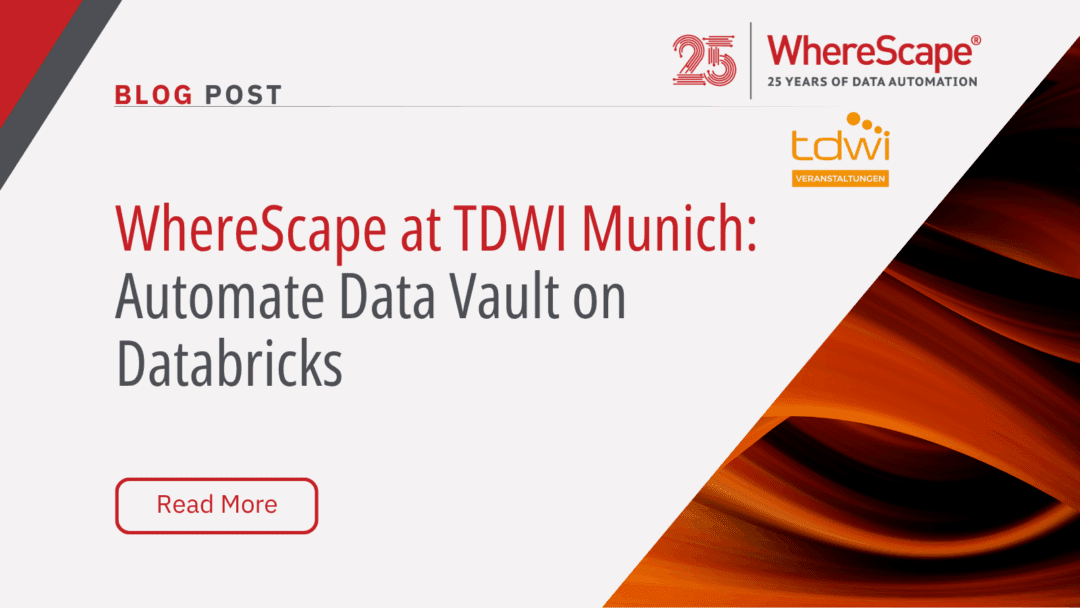
WhereScape at TDWI Munich: Automate Data Vault on Databricks
WhereScape at TDWI Munich 2025: Automate a Full Data Vault on Databricks in Just 45 Minutes June 24–26, 2025 | MOC Munich, Germany As data complexity grows and business demands accelerate, scalable and governed data architectures are no longer optional—they're...
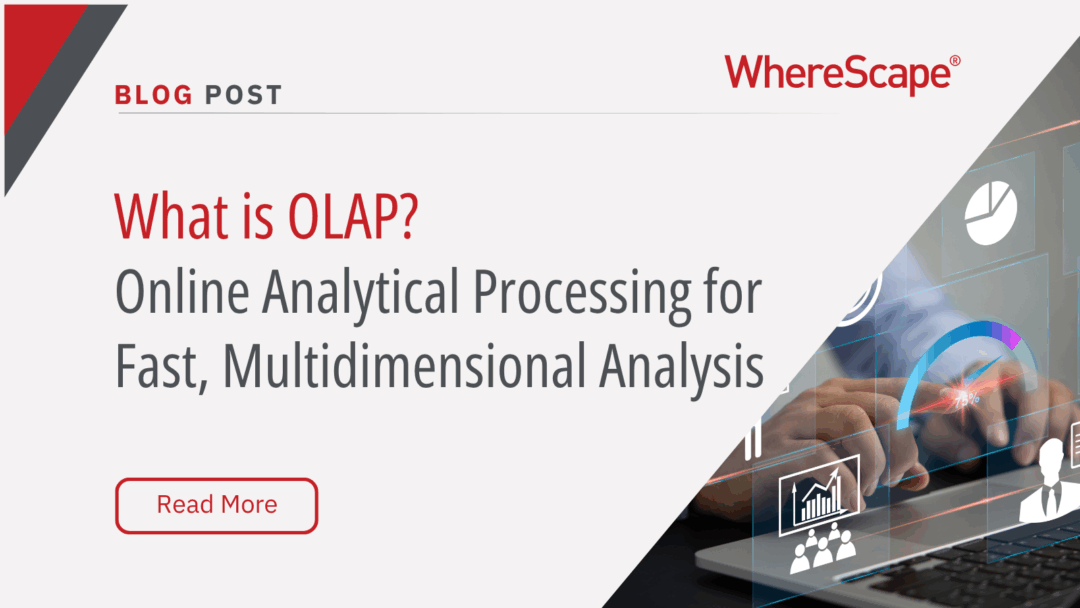
What Is OLAP? Online Analytical Processing for Fast, Multidimensional Analysis
Streamline your data analysis process with OLAP for better business intelligence. Explore the advantages of Online Analytical Processing (OLAP) now! Do you find it challenging to analyze large volumes of data swiftly? A Forrester study reveals that data teams spend...
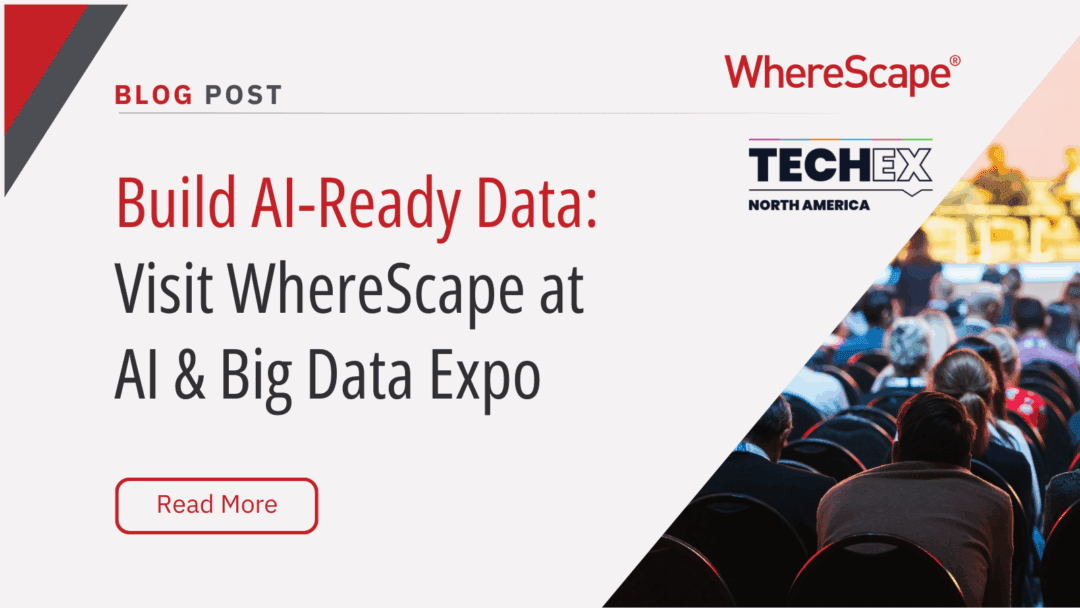
Build AI-Ready Data: Visit WhereScape at AI & Big Data Expo
June 4–5, 2025 | Booth 202 | Santa Clara Convention Center As organizations scale their artificial intelligence and analytics capabilities, the demand for timely, accurate, governed, and AI-ready data has become a strategic priority. According to Gartner, through...
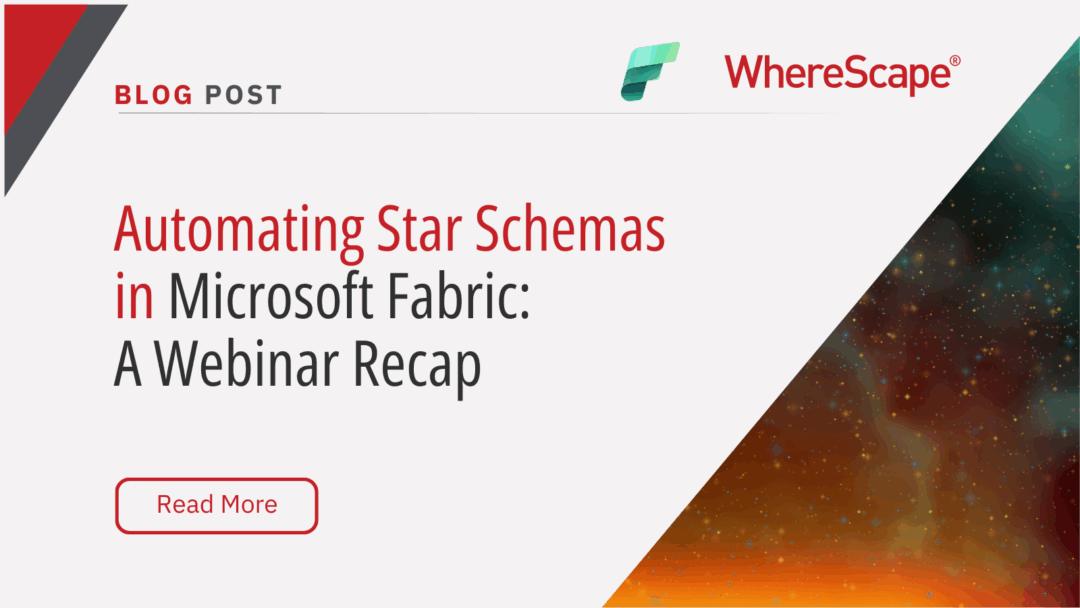
Automating Star Schemas in Microsoft Fabric: A Webinar Recap
From Data Discovery to Deployment—All in One Workflow According to Gartner, data professionals dedicate more than half of their time, 56%, to operational tasks, leaving only 22% for strategic work that drives innovation. This imbalance is especially apparent when...