WhereScape is thrilled to invite you to...
What is the Difference Between a Data Lake and a Data Warehouse?
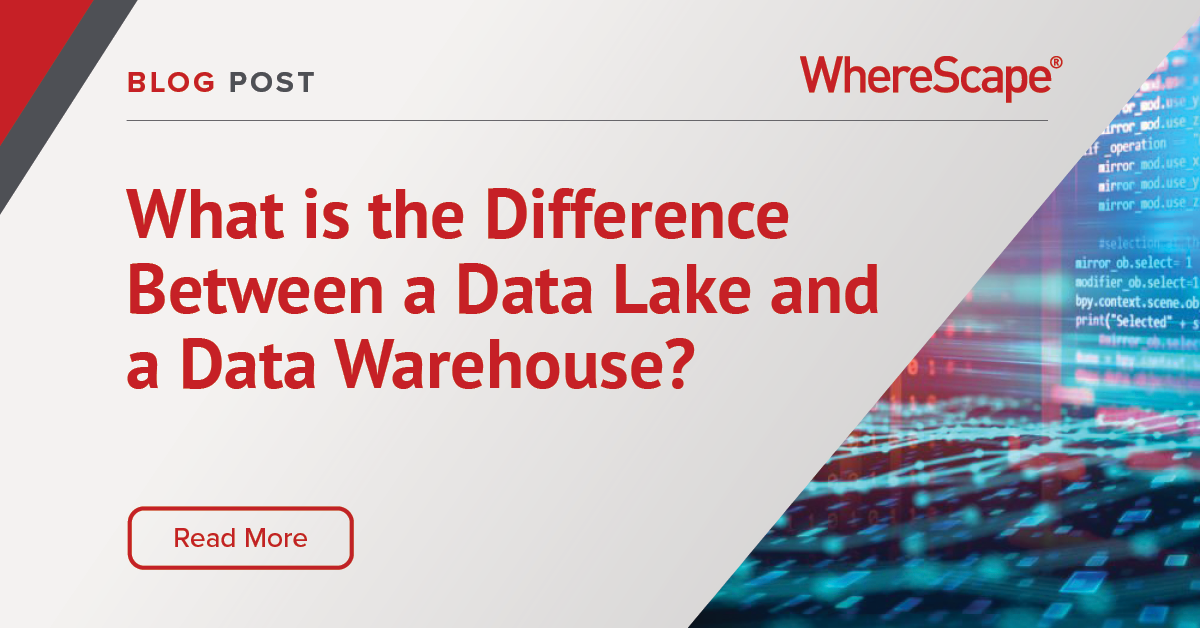
The Data warehouse and data lake are the two leading solutions for enterprise data management. While data warehouses and data lakes might share some overlapping features and use cases, there are fundamental differences in the data management philosophies, design characteristics, and ideal use conditions for each of these platforms.
In this blog post, we take a closer look at the key differences between the data lake and data warehouse platform, and how to choose the right one for your business.
What is a Data Warehouse?
A data warehouse is designed for highly structured data generated by business applications. It brings all your data together and stores it in a structured manner. It is a data management platform that provides business intelligence for structured operational data, usually from a relational database management system (RDBMS). It ingests structured data with predefined schema, then connects that data to downstream analytical tools that support business intelligence (BI) initiatives.
Data warehouses support sequential ETL operations, where data flows in a waterfall model from the raw data format to a fully transformed set, optimized for fast performance. This platform relies on the structure of data to support high-performance SQL (Structured Query Language) operations. Some newer data warehouses support semi-structured data such as JSON, Parquet, and XML files.
It is possible to automate the design, development and production of a data warehouse. Organizations have seen projects estimated to take years reduced to months and sometimes weeks. WhereScape provides data warehouse automation software to achieve these goals.
What is a Data Lake?
A data lake is a centralized data repository where structured, semi-structured, and unstructured data from a variety of sources can be stored in their raw format. It helps eliminate data silos by acting as a single landing zone from multiple sources.
A data lake is ideal for machine learning use cases. It provides SQL-based access to data and native support for programmatic distributed data processing frameworks like Apache Spark and Tensorflow through languages such as Python, Scala, Java, and more. It supports native streaming, where streams of data are processed and made available for analytics as they arrive.
The key purpose of a data lake is to make organizational data from various sources accessible to different end-users like business analysts, data engineers, data scientists, product managers, executives, etc, to leverage insights in a cost-effective manner for improved business performance.
Choosing the right platform for your organization
Both data warehouse and data lake solutions are not mutually exclusive. Neither a data lake nor a data warehouse on its own comprises a data and analytics strategy, but both solutions can be used together.
The data warehouse model is all about functionality and performance. It ingests data from RDBS, transforms it into something useful, then pushes the transformed data to downstream BI and analytics applications. These functions are essential, but the data warehouse paradigm of schema-on-write, tightly coupled storage/compute, and reliance on predefined use cases makes the data warehouse the wrong choice for big, multi-structured data or multi-model capabilities.
In contrast, a data lake is more suited to meeting the demands of a big data world: schema-on-read, loosely coupled storage/compute, and flexible use cases that combine to drive innovation by reducing the time, cost, and complexity of data management. However, without data warehouse functionality, a data lake can become a data swamp.
WhereScape can automate the development and maintenance of your data warehouse. Through two products, WhereScape RED and WhereScape 3D, your organization can achieve its data warehouse goals in a fraction of the time as opposed to developing manually.
If you would like to see WhereScape in action, please request a demo.
What Makes A Really Great Data Model: Essential Criteria And Best Practices
By 2025, over 75% of data models will integrate AI—transforming the way businesses operate. But here's the catch: only those with robust, well-designed data models will reap the benefits. Is your data model ready for the AI revolution?Understanding what makes a great...
Guide to Data Quality: Ensuring Accuracy and Consistency in Your Organization
Why Data Quality Matters Data is only as useful as it is accurate and complete. No matter how many analysis models and data review routines you put into place, your organization can’t truly make data-driven decisions without accurate, relevant, complete, and...
Common Data Quality Challenges and How to Overcome Them
The Importance of Maintaining Data Quality Improving data quality is a top priority for many forward-thinking organizations, and for good reason. Any company making decisions based on data should also invest time and resources into ensuring high data quality. Data...
What is a Cloud Data Warehouse?
As organizations increasingly turn to data-driven decision-making, the demand for cloud data warehouses continues to rise. The cloud data warehouse market is projected to grow significantly, reaching $10.42 billion by 2026 with a compound annual growth rate (CAGR) of...
Developers’ Best Friend: WhereScape Saves Countless Hours
Development teams often struggle with an imbalance between building new features and maintaining existing code. According to studies, up to 75% of a developer's time is spent debugging and fixing code, much of it due to manual processes. This results in 620 million...
Mastering Data Vault Modeling: Architecture, Best Practices, and Essential Tools
What is Data Vault Modeling? To effectively manage large-scale and complex data environments, many data teams turn to Data Vault modeling. This technique provides a highly scalable and flexible architecture that can easily adapt to the growing and changing needs of an...
Scaling Data Warehouses in Education: Strategies for Managing Growing Data Demand
Approximately 74% of educational leaders report that data-driven decision-making enhances institutional performance and helps achieve academic goals. [1] Pinpointing effective data management strategies in education can make a profound impact on learning...
Future-Proofing Manufacturing IT with WhereScape: Driving Efficiency and Innovation
Manufacturing IT strives to conserve resources and add efficiency through the strategic use of data and technology solutions. Toward that end, manufacturing IT teams can drive efficiency and innovation by selecting top tools for data-driven manufacturing and...
The Competitive Advantages of WhereScape
After nearly a quarter-century in the data automation field, WhereScape has established itself as a leader by offering unparalleled capabilities that surpass its competitors. Today we’ll dive into the advantages of WhereScape and highlight why it is the premier data...
Data Management In Healthcare: Streamlining Operations for Improved Care
Appropriate and efficient data management in healthcare plays a large role in staff bandwidth, patient experience, and health outcomes. Healthcare teams require access to patient records and treatment history in order to properly perform their jobs. Operationally,...
Related Content
What Makes A Really Great Data Model: Essential Criteria And Best Practices
By 2025, over 75% of data models will integrate AI—transforming the way businesses operate. But here's the catch: only those with robust, well-designed data models will reap the benefits. Is your data model ready for the AI revolution?Understanding what makes a great...
Guide to Data Quality: Ensuring Accuracy and Consistency in Your Organization
Why Data Quality Matters Data is only as useful as it is accurate and complete. No matter how many analysis models and data review routines you put into place, your organization can’t truly make data-driven decisions without accurate, relevant, complete, and...
Common Data Quality Challenges and How to Overcome Them
The Importance of Maintaining Data Quality Improving data quality is a top priority for many forward-thinking organizations, and for good reason. Any company making decisions based on data should also invest time and resources into ensuring high data quality. Data...
What is a Cloud Data Warehouse?
A cloud data warehouse is an advanced database service managed and hosted over the internet.