WhereScape is thrilled to invite you to...
Why the Ability to Scale is Transformative for Data Teams
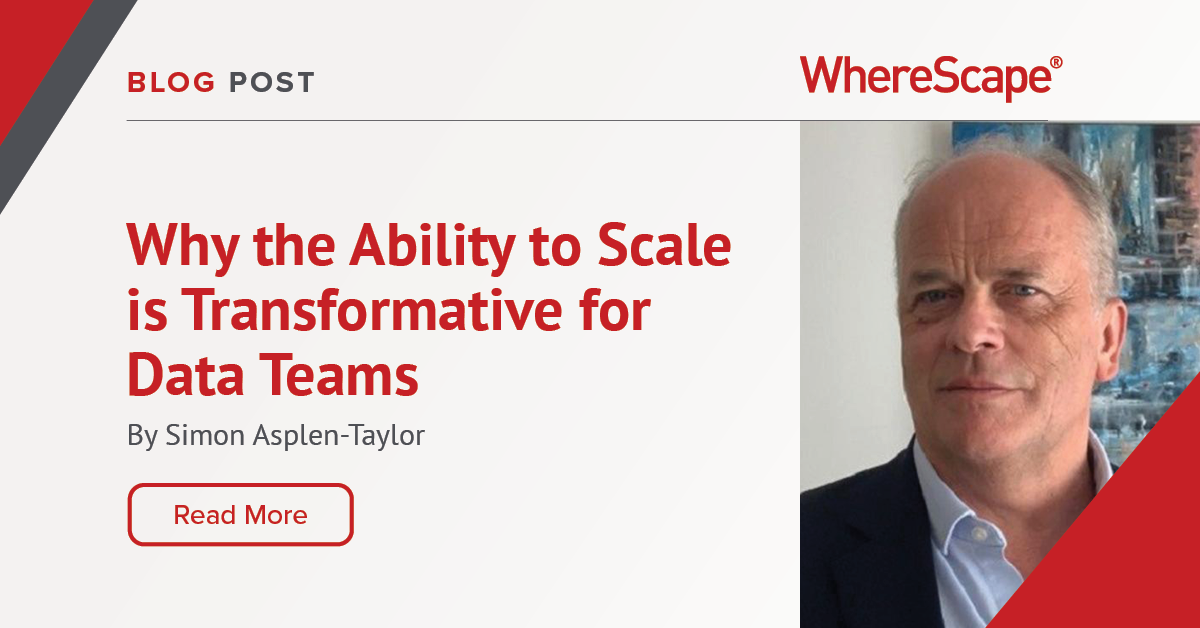
In my previous blog, we talked about quick wins. One of the criteria of a good quick win is that it can be built on or repeated at a later date. That later date has now arrived.
We have to be careful not to take on a myriad of projects that are unrelated to each other. Your data team’s ability to replicate the value achieved in one win with many similar wins across the business offers a potentially huge return on investment. But be careful not to become overwhelmed.
It’s counter-intuitive to think that by starting small you can achieve scale quicker, but this is indeed the case because when you limit your early scope you learn quickly and show tangible results. If you achieve something and it works, you can turn that into widespread board support and funding for a more ambitious project based on the same principles.
If you’ve not experienced that yet, it feels good. Very good.
Tony Robbins, an influential author, motivational speaker and philanthropist says “In business, the definition of “scale” is to increase revenue at a faster rate than costs. Businesses achieve this in a number of ways, from adopting new technologies to finding “gaps” in their operations that can be streamlined. Businesses that are able to add revenue and increase operational demands while maintaining the same costs – or even lowering costs – will be able to scale successfully.” (Robbins Research International, 2021)
This is the thinking that underpins scaling. So you’re ready to scale? There are, effectively, two ways to do this.
Scale Up: Replicate a Defined Project
Some projects make this the natural choice. At an energy company I worked for that ran petrol stations and oil supplies in 23 countries in Africa, our goal was to use data to make the retail offer more efficient. Although Mali is not the same as Burkina Faso, the principle is the same: we planned to capture customer data and use it to find what else the customer might want in their basket based on what most people buy or perhaps seasonal demand. That’s scale: you do one thing and then do it across all 23 countries.
The success of this relies not only on the ability to create quality and trusted data but also on the management task to get all parts of the business to follow the same processes and apply the same standards.
The potential for failure is the absence of those qualities. Certain variations may occur between the different places in the business where the project is implemented, and data acquisition may vary, as might management ability. This may create governance challenges. Also, there may be variation in laws governing the acquisition, sharing and use of data, and in the habits and norms in the two places.
Scale Out: Find an Adjacent Activity
This may be more challenging. The data capability grants an opportunity to innovate across the business. A Single Customer View (SCV) is useful to deepen existing relationships with customers – buying the same thing more often, but also to develop new products and services that they buy. The potential is that, in data terms, you can use the same SCV in an entirely new way.
There are two caveats: The first is that this may require new, or more, data. For example, some external data that tells us more about the customer’s habits when they are not transacting with our business. The second is that it may require new ways of working. That means cooperation between two business units that until now worked separately or discipline in how to execute a marketing plan based on knowledge rather than activity. For this, you rely on the willingness of the managers in those parts of the business to evangelize data and change ingrained habits. So it’s not entirely straightforward, but this is a huge step towards establishing a data-driven culture, which is our ultimate goal.
What to do Once you Scale Successfully
So now you’ve been the leader of a successful data transformation project, you will have no shortage of people who will want to support you because you are successful. This may present us with a problem: you have limited time in your day and too many things to do already. This means that scaling up isn’t about suddenly doing everything, everywhere, all the time, for everyone who sends you an email. Scaling is about putting in place ways to achieve a maximum return on the data team’s investment.
This is an important aspect of scaling up. From the outside, everyone can see the similarities between what you have just done and what they want for their bit of the business. Look closer, and they often overestimate the regularities. If something is a bit like something else, if there are a few aspects of that project that can be carried over into the next one, it’s not scaling up, it’s an entirely new piece of work.
If you’re going through this, you probably have requests to replicate projects on your desk already. It is important that you evaluate the need, the prospect of success, and the constraints on delivery that you will encounter. You have to learn to say no and have the confidence and backing to do that. Achieve this and it’ll be a major breakthrough for your data team.
Summary
- Having created success in one part of the business, the ability to replicate this in other areas or processes offers a potentially enormous return on investment.
- The knowledge that the data team has of these processes is an important factor in applying them quickly and effectively.
- You can crowdsource ideas for scaling using techniques like hackathons, bringing together your whole data community.
- These also help to establish the importance of the data transformation, because scaling will make your work more visible.
- Implementation requires the cooperation of the business, and especially of allies inside it if it is to happen at speed.
What Makes A Really Great Data Model: Essential Criteria And Best Practices
By 2025, over 75% of data models will integrate AI—transforming the way businesses operate. But here's the catch: only those with robust, well-designed data models will reap the benefits. Is your data model ready for the AI revolution?Understanding what makes a great...
Guide to Data Quality: Ensuring Accuracy and Consistency in Your Organization
Why Data Quality Matters Data is only as useful as it is accurate and complete. No matter how many analysis models and data review routines you put into place, your organization can’t truly make data-driven decisions without accurate, relevant, complete, and...
Common Data Quality Challenges and How to Overcome Them
The Importance of Maintaining Data Quality Improving data quality is a top priority for many forward-thinking organizations, and for good reason. Any company making decisions based on data should also invest time and resources into ensuring high data quality. Data...
What is a Cloud Data Warehouse?
As organizations increasingly turn to data-driven decision-making, the demand for cloud data warehouses continues to rise. The cloud data warehouse market is projected to grow significantly, reaching $10.42 billion by 2026 with a compound annual growth rate (CAGR) of...
Developers’ Best Friend: WhereScape Saves Countless Hours
Development teams often struggle with an imbalance between building new features and maintaining existing code. According to studies, up to 75% of a developer's time is spent debugging and fixing code, much of it due to manual processes. This results in 620 million...
Mastering Data Vault Modeling: Architecture, Best Practices, and Essential Tools
What is Data Vault Modeling? To effectively manage large-scale and complex data environments, many data teams turn to Data Vault modeling. This technique provides a highly scalable and flexible architecture that can easily adapt to the growing and changing needs of an...
Scaling Data Warehouses in Education: Strategies for Managing Growing Data Demand
Approximately 74% of educational leaders report that data-driven decision-making enhances institutional performance and helps achieve academic goals. [1] Pinpointing effective data management strategies in education can make a profound impact on learning...
Future-Proofing Manufacturing IT with WhereScape: Driving Efficiency and Innovation
Manufacturing IT strives to conserve resources and add efficiency through the strategic use of data and technology solutions. Toward that end, manufacturing IT teams can drive efficiency and innovation by selecting top tools for data-driven manufacturing and...
The Competitive Advantages of WhereScape
After nearly a quarter-century in the data automation field, WhereScape has established itself as a leader by offering unparalleled capabilities that surpass its competitors. Today we’ll dive into the advantages of WhereScape and highlight why it is the premier data...
Data Management In Healthcare: Streamlining Operations for Improved Care
Appropriate and efficient data management in healthcare plays a large role in staff bandwidth, patient experience, and health outcomes. Healthcare teams require access to patient records and treatment history in order to properly perform their jobs. Operationally,...
Related Content
What Makes A Really Great Data Model: Essential Criteria And Best Practices
By 2025, over 75% of data models will integrate AI—transforming the way businesses operate. But here's the catch: only those with robust, well-designed data models will reap the benefits. Is your data model ready for the AI revolution?Understanding what makes a great...
Guide to Data Quality: Ensuring Accuracy and Consistency in Your Organization
Why Data Quality Matters Data is only as useful as it is accurate and complete. No matter how many analysis models and data review routines you put into place, your organization can’t truly make data-driven decisions without accurate, relevant, complete, and...
Common Data Quality Challenges and How to Overcome Them
The Importance of Maintaining Data Quality Improving data quality is a top priority for many forward-thinking organizations, and for good reason. Any company making decisions based on data should also invest time and resources into ensuring high data quality. Data...
What is a Cloud Data Warehouse?
A cloud data warehouse is an advanced database service managed and hosted over the internet.