Join us for a 90-minute, insightful webinar...
Gartner’s Report on Data Hubs, Lakes & Warehouses Highlights Knowledge Gap for Data Managers
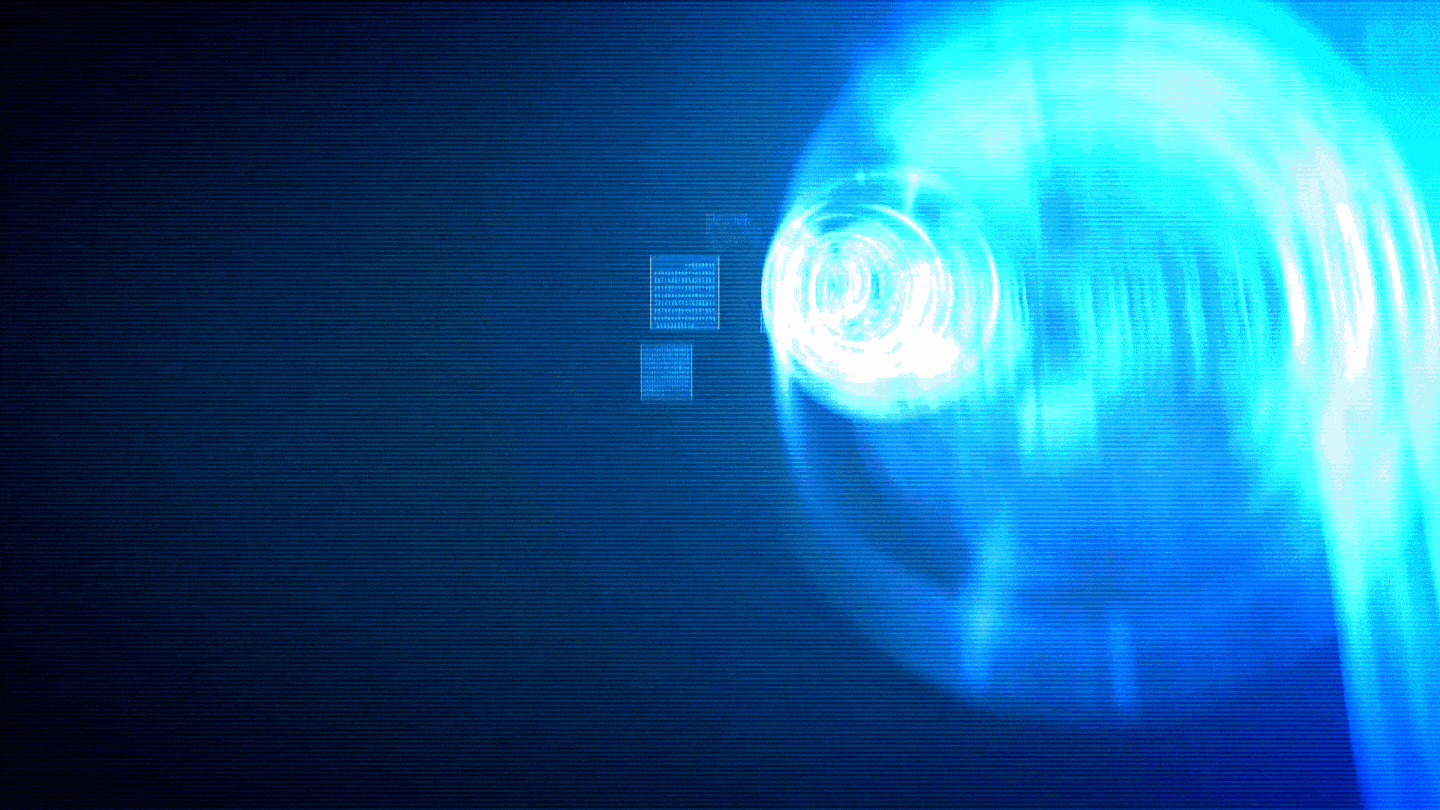
Gartner’s paper, Data Hubs, Data Lakes and Data Warehouses: How They Are Different and Why They Are Better Together, serves as much as a cautionary piece as an informative one. Based on inquiries made to the analyst firm over the past few years, is it apparent that a real gap in knowledge exists when it comes to what these three data structures do and how they should be employed.
“For example, while Gartner client inquiries referring to data hubs increased by 20% from 2018 through 2019, more than 25% of these inquiries were actually about data lake concepts.” (Data Hubs, Data Lakes and Data Warehouses: How They Are Different and Why They Are Better Together by Ted Friedman, Nick Heudecker.)
At the time of the report’s publication in 2020, the percentage of companies using data hubs, lakes and warehouses looked like this:
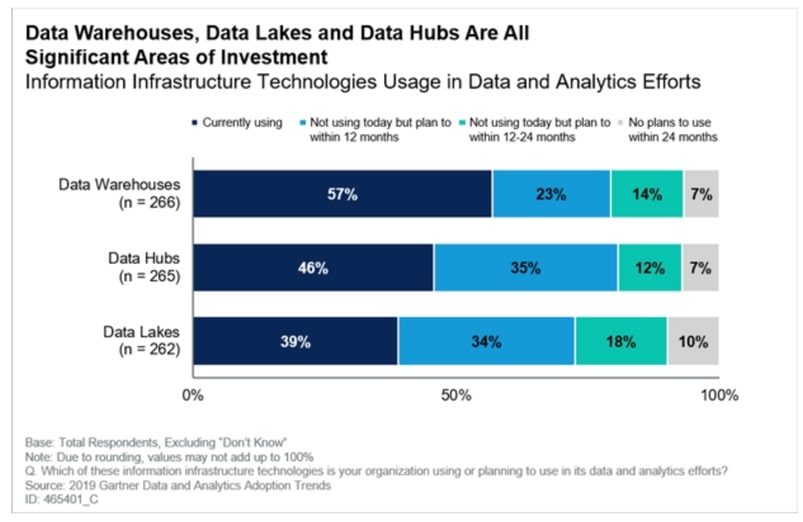
With many companies using all three of these structures already, it’s no exaggeration to say how well companies can understand and harness the potential of data lakes, warehouses and hubs can and will shape their success. This explains the urgency underlying this piece; at present, huge investments are being made by many people who either do not fully understand what each of these three entities does alone and/or how they can be combined most effectively.
How Data Warehouses, Lakes and Hubs Work
Data Warehouses should be used for the analysis of structured data, Data Lakes for analysis of unstructured or semi-structured data, and Data Hubs for communicating the resultant BI to the people who need to act on it. However, many mistakenly think that these three entities do the same thing in different ways, and so are interchangeable. It’s important that business leaders not only understand this for themselves but communicate it throughout the company to democratize the use of data.
Data Lakes and the exploratory technologies that unstructured big data enables are only as useful as your company’s ability to assimilate their findings into a structured environment. This is where the Data Warehouse takes over: a Data Lake can be added as a source to a Data Warehouse, and its data blended with other real-time and batch sources to provide rich, contextualized business insight. Read more on Data Lakehouses here.
Of the three structures, it is ironic that the one managers need to know best is the least understood. The Data Hub is where BI is not only shared but is also available for governance by those responsible for it. As its name suggests a hub also “enables data flow between diverse endpoints”
One of the main recommendations of Gartner’s report is to: “Maximize your ability to support a broader range of diverse use cases by identifying the ways that these structures can be used in combination. For example, data can be delivered to analytic structures (Data Warehouses and Data Lakes) using a Data Hub as a point of mediation and governance.” (Data Hubs, Data Lakes and Data Warehouses: How They Are Different and Why They Are Better Together by Ted Friedman, Nick Heudecker.)
Dealing with Disruption
The report also highlights the need to be agile in how your company can ingest new data from various sources in different formats. Those that can, are able to adapt to disruption and monetize it before their competitors. This supports the use of both a Data Warehouse and lake in conjunction as part of a logical Data Warehouse, and also of an end-to-end automated infrastructure to manage and change it quickly as needed.
While the exponential growth of data makes more insight available, it also means the infrastructure that stores and analyses it becomes necessarily more complex. This infrastructure needs to adapt as new demands emerge (constantly) and as data sources evolve (periodically). It’s a fallacy to think we can create the ultimate data infrastructure that won’t need to be changed.
The Dangers of Ambiguity
Perhaps by reading this piece, data leaders can iron out any ambiguity and potentially make their companies more successful. Misunderstanding also has internal implications in that expectations and reality can be quite different if those leading the data department have different definitions of certain infrastructure than those building and using it day-to-day.
The report is vital reading for data leaders who have even a hint of doubt in their minds of the purpose and role of the Data Warehouse, lake or hub. It could mean the difference between a successful data project, or a failed one in which the roles of the various technologies and staff are not clearly defined.
Optimizing Enterprise Data Management Solutions with WhereScape RED
Empowering Enterprise Data Management with WhereScape RED Choosing the best data warehouse automation software can make enterprises more scalable, accurate, and competitive. WhereScape RED is one of the most empowering enterprise data management solutions available,...
Enhancing Data Interoperability: Connecting Diverse Systems with WhereScape
Understanding Data Interoperability in Modern Enterprises Modern enterprises use countless different systems to gather, store, analyze, and process data. From varying data types to unique purposes and levels of security, a single enterprise handles data in several...
Gartner Highlights the Rise of Data Warehouse Automation
Imagine a world where the manual, tedious tasks of data warehouse development are a thing of the past. This isn't a far-off fantasy but a present-day reality, thanks to advances in Data Warehouse Automation (DWA). Gartner's latest report by analyst Henry Cook,...
Experience the Power of WhereScape 3D 9.0.3: New Features and Improvements
We’re thrilled to introduce our latest iteration of WhereScape 3D! Version 9.0.3 brings a host of new features and enhancements designed to make your data warehousing journey smoother, faster, and more efficient. Let’s dive into the details of what you can expect from...
Ahead of the Curve: Future Trends in Data Automation and WhereScape’s Pioneering Solutions
The Evolving Landscape of Data Automation As new technologies emerge and existing tools constantly change and improve, the world of data automation transforms rapidly. Even the most well-versed data teams find themselves disoriented and overwhelmed in the face of...
Investing in Data Automation: A Strategic Approach to Business Growth
Unlocking Growth: The Strategic Advantage of Data Automation Organizations reaping the benefits of data automation stay ahead of industry trends and improve the efficiency of their operations and decision-making. Data automation tools offer a strategic advantage for...
Data + AI Summit 2024: Key Takeaways and Innovations
The Data + AI Summit 2024, hosted by Databricks at the bustling Moscone Center in San Francisco, has concluded with remarkable revelations and forward-looking innovations. Drawing over 16,000 attendees in person and virtually connecting over 60,000 participants from...
WhereScape RED 10.1 is Here: Enhanced Scheduling and Customization
We’re proud to announce the highly anticipated WhereScape RED 10.1 is now available, and it’s packed with exciting new features and enhancements designed to make your data warehousing experience more efficient and enjoyable. Let's take a closer look at what’s new and...
Supercharging Data Integration: The WhereScape and Databricks Advantage
The demand for robust data management systems has never been higher, and Databricks has quickly become a favored choice for cloud-based solutions. Its powerful capabilities make it a top contender for managing large-scale data, but when combined with WhereScape's...
Empowering Customer Success: WhereScape’s Comprehensive Support and Training Resources
Enhancing Operational Success with WhereScape’s Support Systems At WhereScape, we understand that a data warehouse is only useful to the extent that it is understood. In order to drive your organization closer to your key goals and objectives, you need full mastery of...
Related Content
Optimizing Enterprise Data Management Solutions with WhereScape RED
Empowering Enterprise Data Management with WhereScape RED Choosing the best data warehouse automation software can make enterprises more scalable, accurate, and competitive. WhereScape RED is one of the most empowering enterprise data management solutions available,...
Enhancing Data Interoperability: Connecting Diverse Systems with WhereScape
Understanding Data Interoperability in Modern Enterprises Modern enterprises use countless different systems to gather, store, analyze, and process data. From varying data types to unique purposes and levels of security, a single enterprise handles data in several...
Gartner Highlights the Rise of Data Warehouse Automation
Imagine a world where the manual, tedious tasks of data warehouse development are a thing of the past. This isn't a far-off fantasy but a present-day reality, thanks to advances in Data Warehouse Automation (DWA). Gartner's latest report by analyst Henry Cook,...
Experience the Power of WhereScape 3D 9.0.3: New Features and Improvements
We’re thrilled to introduce our latest iteration of WhereScape 3D! Version 9.0.3 brings a host of new features and enhancements designed to make your data warehousing journey smoother, faster, and more efficient. Let’s dive into the details of what you can expect from...