Tune in for a free, live virtual hands-on lab...
The Assembly Line for Your Data: How Automation Transforms Data Projects
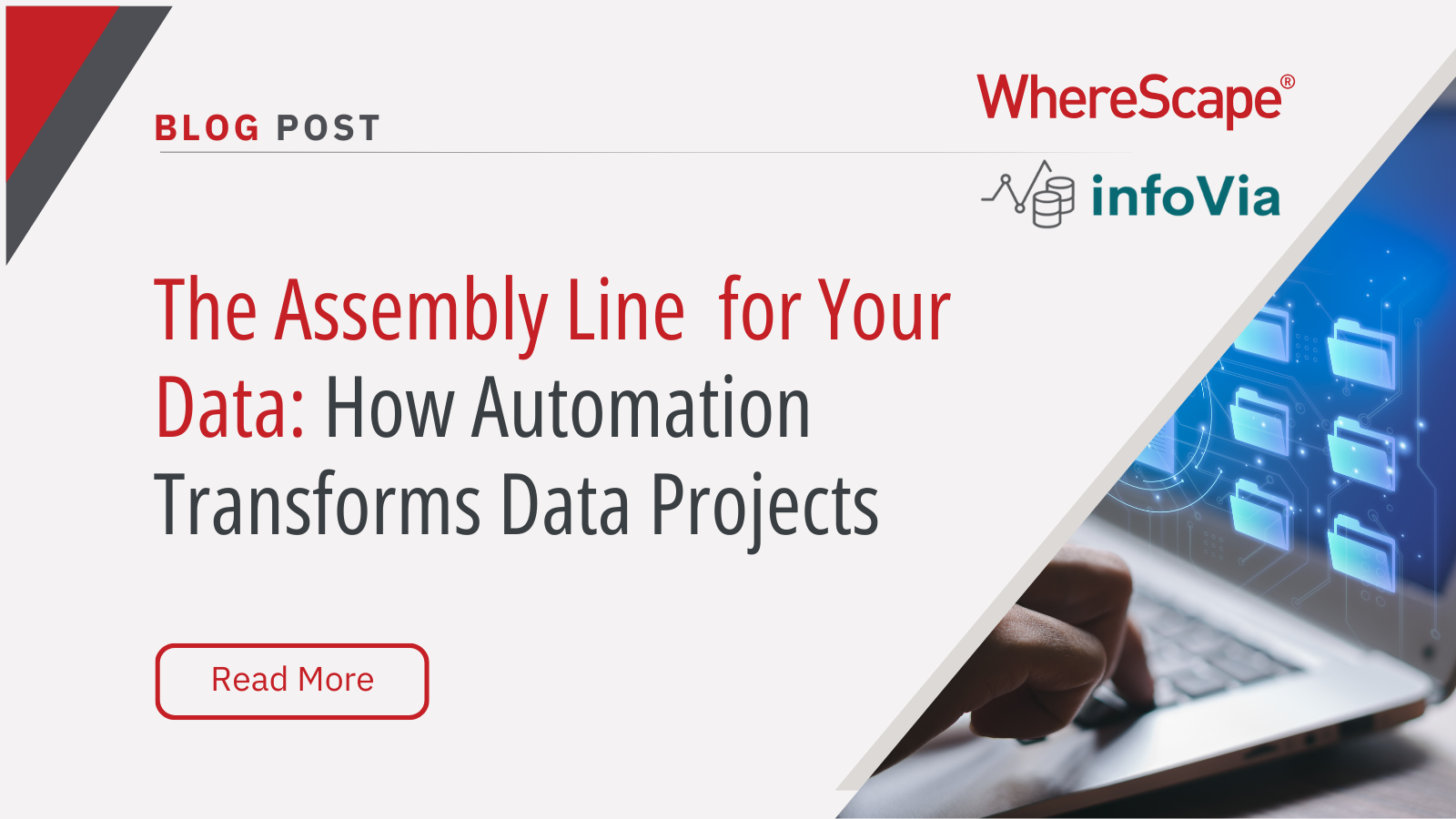
Imagine an old-fashioned assembly line. Workers pass components down the line, each adding their own piece. It’s repetitive, prone to errors, and can grind to a halt if one person falls behind. Now, picture the modern version—robots assembling products with speed, precision, and adaptability. This is the transformation automation brings to data projects.
Data isn’t just numbers; it’s the raw material of decision-making. Yet, for many organizations, managing it feels like an outdated assembly line—manual, slow, and full of bottlenecks. What if you could replace that with a streamlined, efficient system that scales with your needs? Welcome to the world of data automation.
Why Automation Matters in Data Projects
Gartner estimates that 87% of data science projects fail to reach production. That’s like designing a car that never makes it to the showroom. Automation provides the assembly line your data projects need to move from concept to delivery efficiently.
Here’s how:
- Reduces Manual Labor: Automation eliminates repetitive tasks like code generation and data validation, reducing errors and freeing up teams to focus on strategic initiatives.
- Improves Scalability: As your data grows, automation ensures workflows keep pace without reinventing the wheel.
- Enhances Agility: Tools like WhereScape empowers teams to prototype and iterate quickly, delivering insights faster.
Building Your Data Automation Assembly Line
Data Automation isn’t magic—it’s methodical. It requires a clear roadmap, just like building a state-of-the-art production line. Here’s how to get started:
1. Assess the Current State
Think of your tools and processes as your raw materials. Are they helping or hindering? Involve stakeholders early to identify gaps and align on goals.
2. Define the Blueprint
Set measurable objectives, such as improving data quality or reducing project timelines. Prioritize areas where automation will have the biggest business impact.
3. Pick the Right Machinery
Choose tools that can scale and adapt, like WhereScape 3D for modeling and WhereScape RED for automating code. Metadata-driven solutions are your blueprint for consistency and accuracy.
4. Strategize Like a Factory Manager
Break your roadmap into phases with clear milestones. Start with high-value quick wins, like automating data validation or reporting, to prove ROI early.
5. Implement Governance and Quality Control
Data is only valuable if it’s reliable. Use rigorous validation, monitoring, and security protocols to keep your “product” intact.
6. Partner with Automation Experts
Just as automakers rely on specialized engineers, partner with experts like infoVia. Their metadata-first strategies ensure seamless integration, scalability, and governance.
Avoiding Common Pitfalls
Automation is a tool, not a cure-all. To succeed:
- Start Small: Target low-hanging fruit with clear ROI.
- Avoid Overengineering: Focus on solving business challenges, not creating complexity.
- Optimize Continuously: Regularly refine your processes to stay efficient and effective.
The Power of Tools and Expertise
Think of WhereScape and infoVia as the robotics and engineers of your assembly line.
- WhereScape 3D: Maps out your data like a CAD model for a car.
- WhereScape RED: Automates repetitive coding, letting your team focus on innovation.
- infoVia: Provides expert guidance to align tools, teams, and goals seamlessly.
By combining the right tools with expert guidance, your data projects will deliver insights faster, more accurately, and with less effort.
Modernizing your data projects isn’t a luxury; it’s a necessity. Automation is the assembly line that transforms raw data into actionable insights. By building a roadmap, leveraging tools like WhereScape, and partnering with experts like infoVia, you’ll future-proof your data strategy and drive business results.
Ready to streamline your data production line? Contact infoVia today to take the first step toward data automation excellence.
WhereScape at TDWI Munich: Automate Data Vault on Databricks
WhereScape at TDWI Munich 2025: Automate a Full Data Vault on Databricks in Just 45 Minutes June 24–26, 2025 | MOC Munich, Germany As data complexity grows and business demands accelerate, scalable and governed data architectures are no longer optional—they're...
What Is OLAP? Online Analytical Processing for Fast, Multidimensional Analysis
Streamline your data analysis process with OLAP for better business intelligence. Explore the advantages of Online Analytical Processing (OLAP) now! Do you find it challenging to analyze large volumes of data swiftly? A Forrester study reveals that data teams spend...
Build AI-Ready Data: Visit WhereScape at AI & Big Data Expo
June 4–5, 2025 | Booth 202 | Santa Clara Convention Center As organizations scale their artificial intelligence and analytics capabilities, the demand for timely, accurate, governed, and AI-ready data has become a strategic priority. According to Gartner, through...
Automating Star Schemas in Microsoft Fabric: A Webinar Recap
From Data Discovery to Deployment—All in One Workflow According to Gartner, data professionals dedicate more than half of their time, 56%, to operational tasks, leaving only 22% for strategic work that drives innovation. This imbalance is especially apparent when...
What is a Data Model? How Structured Data Drives AI Success
What is a data model? According to the 2020 State of Data Science report by Anaconda, data scientists spend about 45% of their time on data preparation tasks, including cleaning and loading data. Without well-structured data, even the most advanced AI systems can...
ETL vs ELT: What are the Differences?
In working with hundreds of data teams through WhereScape’s automation platform, we’ve seen this debate evolve as businesses modernize their infrastructure. Each method, ETL vs ELT, offers a unique pathway for transferring raw data into a warehouse, where it can be...
Dimensional Modeling for Machine Learning
Kimball’s dimensional modeling continues to play a critical role in machine learning and data science outcomes, as outlined in the Kimball Group’s 10 Essential Rules of Dimensional Modeling, a framework still widely applied in modern data workflows. In a recent...
Automating Data Vault in Databricks | WhereScape Recap
Automating Data Vault in Databricks can reduce time-to-value by up to 70%—and that’s why we hosted a recent WhereScape webinar to show exactly how. At WhereScape, modern data teams shouldn't have to choose between agility and governance. That's why we hosted a live...
WhereScape Recap: Highlights From Big Data & AI World London 2025
Big Data & AI World London 2025 brought together thousands of data and AI professionals at ExCeL London—and WhereScape was right in the middle of the action. With automation taking center stage across the industry, it was no surprise that our booth and sessions...
Why WhereScape is the Leading Solution for Healthcare Data Automation
Optimizing Healthcare Data Management with Automation Healthcare organizations manage vast amounts of medical data across EHR systems, billing platforms, clinical research, and operational analytics. However, healthcare data integration remains a challenge due to...
Related Content
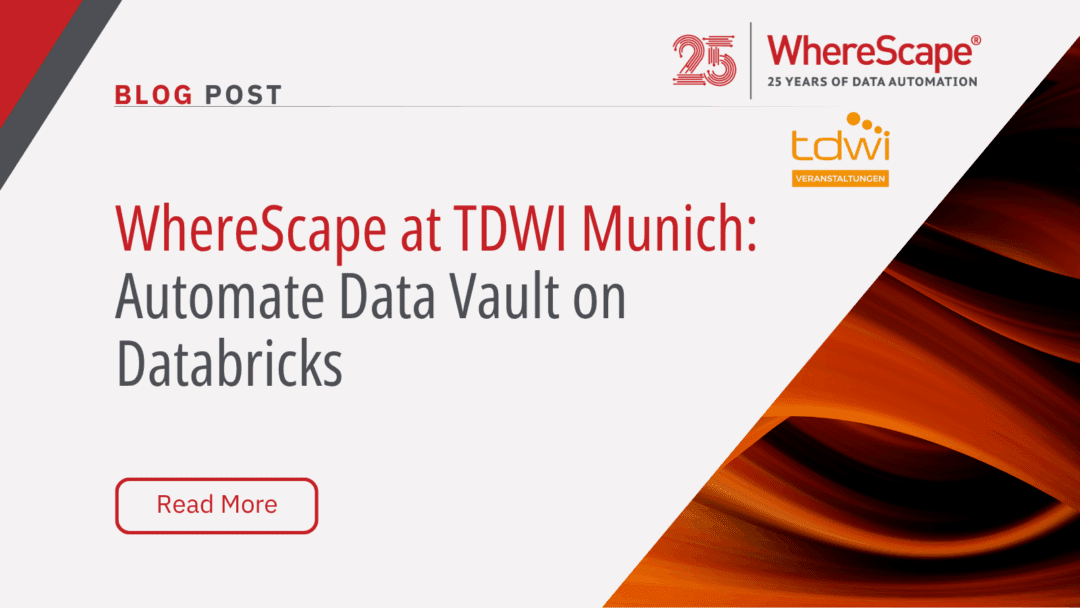
WhereScape at TDWI Munich: Automate Data Vault on Databricks
WhereScape at TDWI Munich 2025: Automate a Full Data Vault on Databricks in Just 45 Minutes June 24–26, 2025 | MOC Munich, Germany As data complexity grows and business demands accelerate, scalable and governed data architectures are no longer optional—they're...
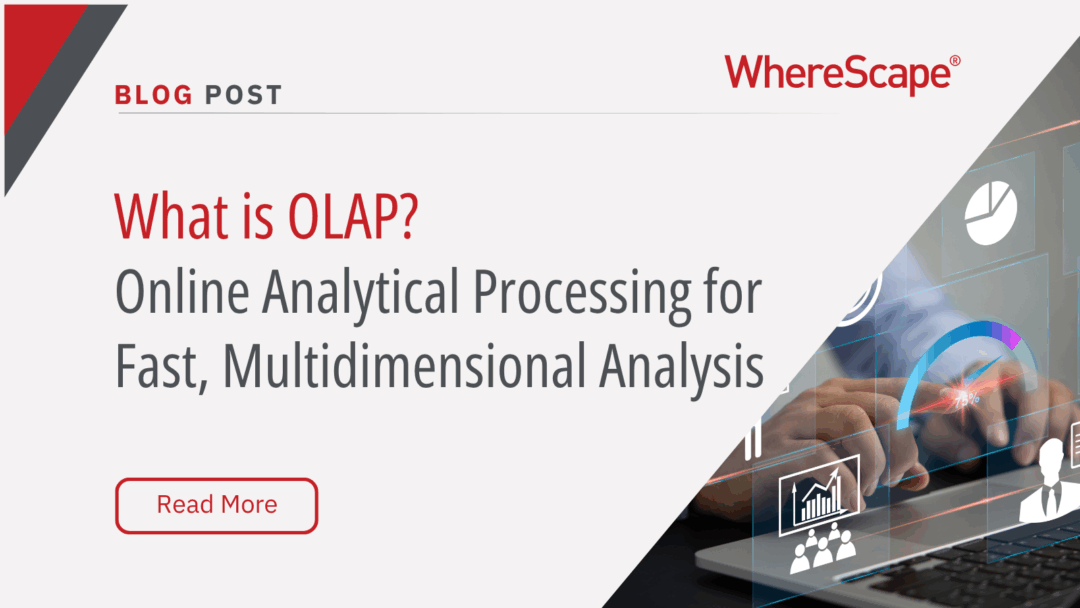
What Is OLAP? Online Analytical Processing for Fast, Multidimensional Analysis
Streamline your data analysis process with OLAP for better business intelligence. Explore the advantages of Online Analytical Processing (OLAP) now! Do you find it challenging to analyze large volumes of data swiftly? A Forrester study reveals that data teams spend...
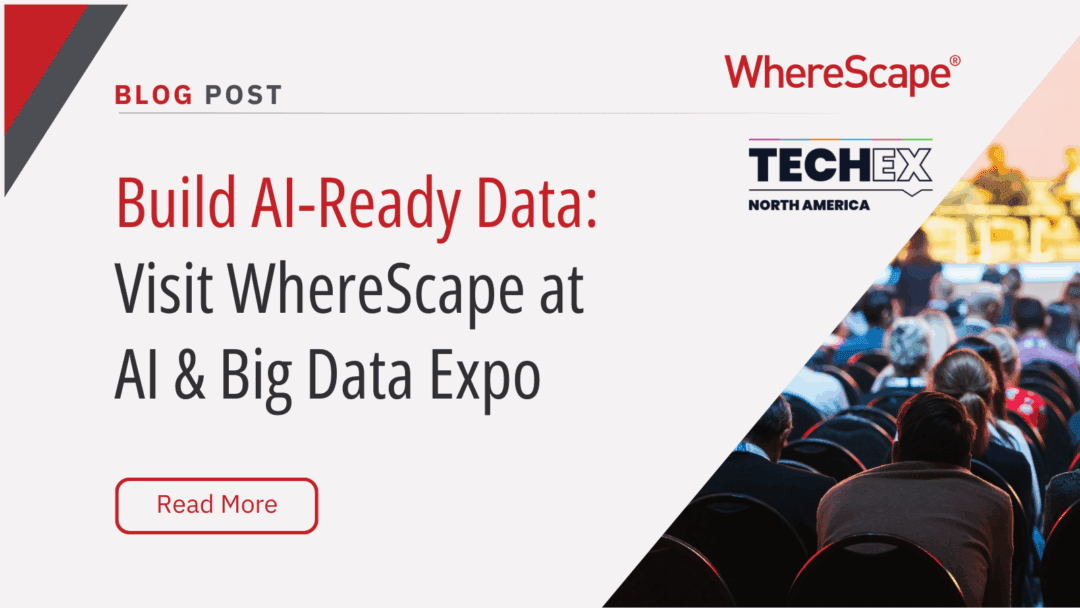
Build AI-Ready Data: Visit WhereScape at AI & Big Data Expo
June 4–5, 2025 | Booth 202 | Santa Clara Convention Center As organizations scale their artificial intelligence and analytics capabilities, the demand for timely, accurate, governed, and AI-ready data has become a strategic priority. According to Gartner, through...
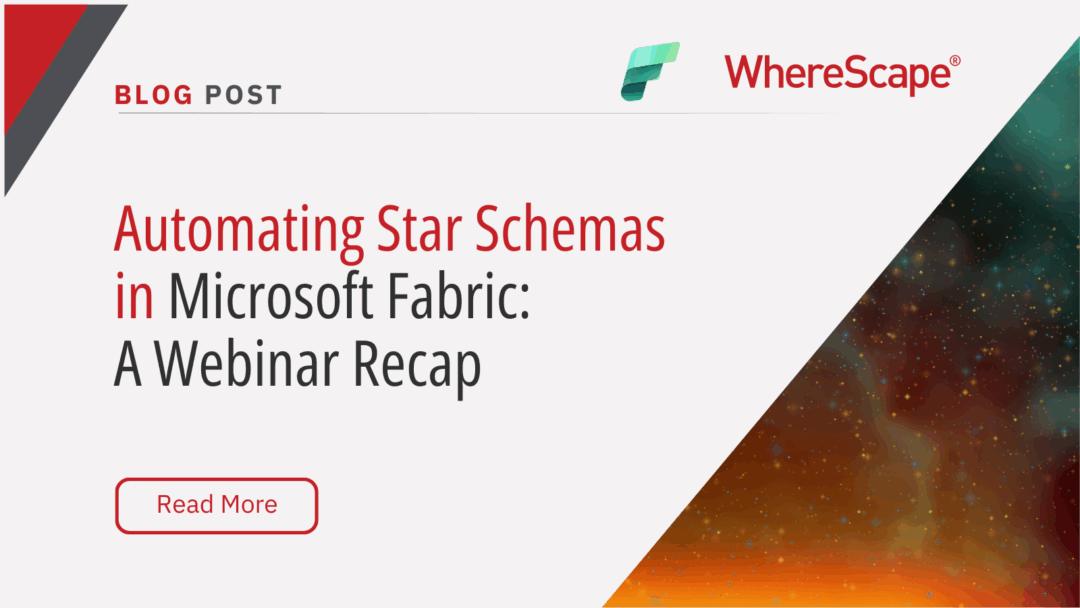
Automating Star Schemas in Microsoft Fabric: A Webinar Recap
From Data Discovery to Deployment—All in One Workflow According to Gartner, data professionals dedicate more than half of their time, 56%, to operational tasks, leaving only 22% for strategic work that drives innovation. This imbalance is especially apparent when...