Join our fast-and-friendly virtual tour to...
Want Better AI Data Management? Data Automation is the Answer
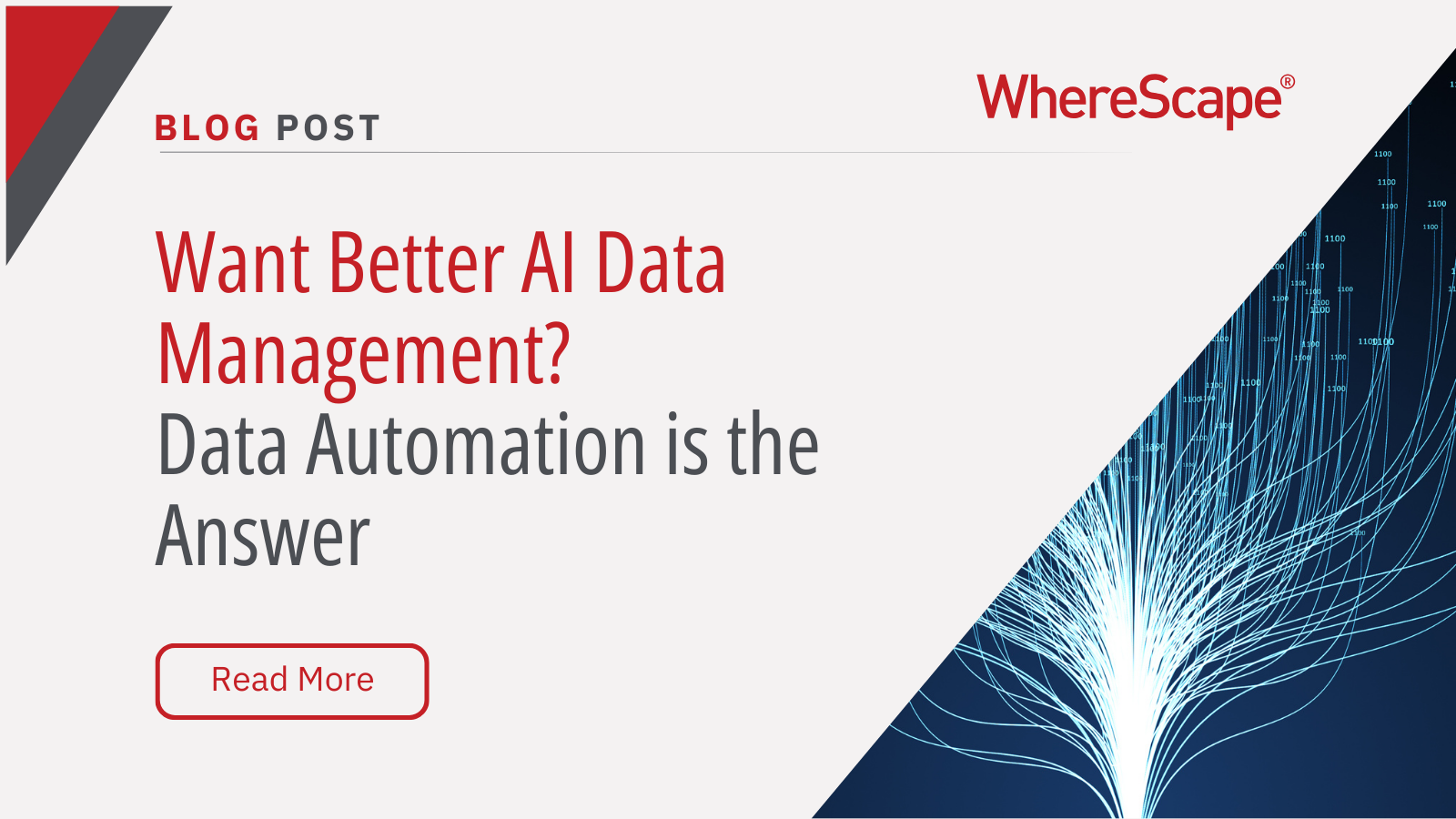
Understanding the AI Landscape
Imagine losing 6% of your annual revenue—simply due to poor data quality. A recent survey found that underperforming AI models, built using low-quality or inaccurate data, cost companies an average of $406 million annually.
Artificial intelligence (AI) is no longer a futuristic concept—it is an integral part of modern business operations much as moving to the cloud is. From automating tasks and optimizing workflows to providing deep analytical insights, AI and AI data management is revolutionizing industries at an unprecedented pace. However, AI solutions rely on effective data management, data validation, and AI data governance.
AI delivers accurate, reliable, and unbiased results when built on a robust data management framework. Structured, high-quality data optimizes AI model performance. Data modeling plays a crucial role by providing a structured blueprint that organizes data, ensures accessibility, and enhances its meaning for AI applications.
The Role of Data Modeling in AI Data Management
Data modeling is the foundation of AI data management, defining how data is structured, stored, and utilized within an AI system. A well-designed data model enables:
- High-quality data ingestion – Ensuring AI systems receive clean, well-structured data.
- Efficient data processing – Reducing redundancy and improving data retrieval speed.
- Interoperability – Facilitating seamless integration between AI models and diverse data sources.
- Scalability – Allowing AI models to adapt to growing datasets without performance degradation.
Modern data modeling tools, such as WhereScape, automate and streamline these processes, ensuring that businesses can implement AI with minimal friction.
Industries Embracing AI Today
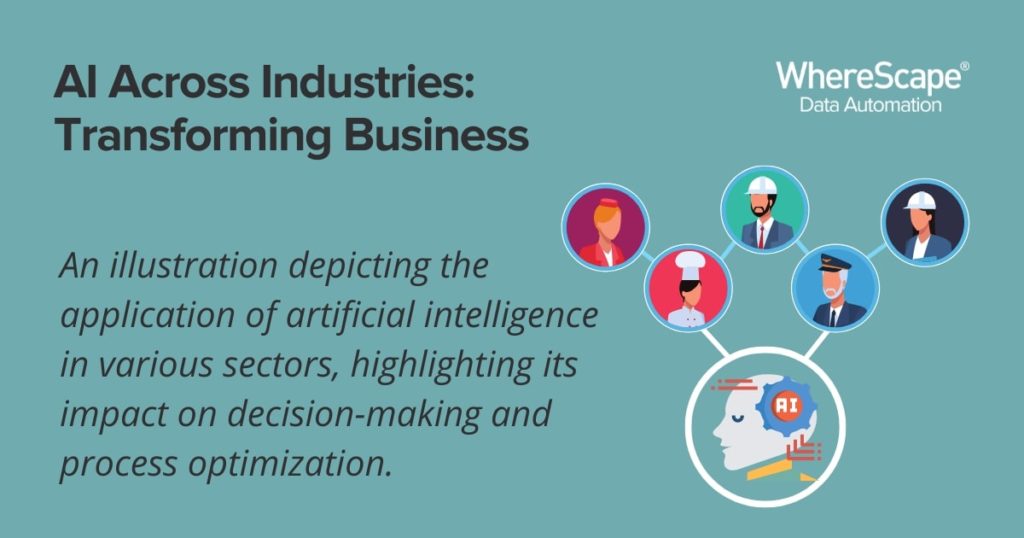
Artificial intelligence (AI) has rapidly become a transformative technology in many industries. Below are some sectors where the application of AI is already prevalent:
- Healthcare: AI is used for predictive analytics, patient monitoring, disease diagnosis, drug discovery, and personalization of treatment plans. AI algorithms can also analyze medical imaging to detect anomalies and conditions.
- Retail: AI helps in predictive inventory management, personalized marketing, customer service through chatbots, and product recommendations. It also aids in analyzing consumer behavior patterns.
- Finance and Banking: AI is used in fraud detection, risk assessment, financial planning, algorithmic trading, and customer service automation. It also assists in regulatory compliance and document processing.
- Manufacturing: AI optimizes supply chains, improves quality control, enables predictive maintenance, and enhances safety. It also assists with automation and robotics in the assembly line.
- Transportation and Logistics: Autonomous vehicles, route optimization, demand forecasting, and tracking and monitoring systems use AI. It’s also applied in drone technology for deliveries.
- Agriculture: AI aids in precision farming, crop and soil monitoring, predictive analysis for weather and crop yield, and automation of irrigation systems.
- Education: AI is applied in personalized learning, automation of administrative tasks, intelligent tutoring systems, and adaptive learning technologies.
- Energy: AI optimizes energy usage, predicts equipment failures, and enables intelligent grids. It also assists in automating drilling and exploration in the oil and gas industry.
- Media and Entertainment: AI is used in content recommendations, optimizing user experience, advertising, personalizing content, and creating content.
- Real Estate: AI is used for property recommendations based on user preferences, estimating property value, virtual property tours, and automating lease management.
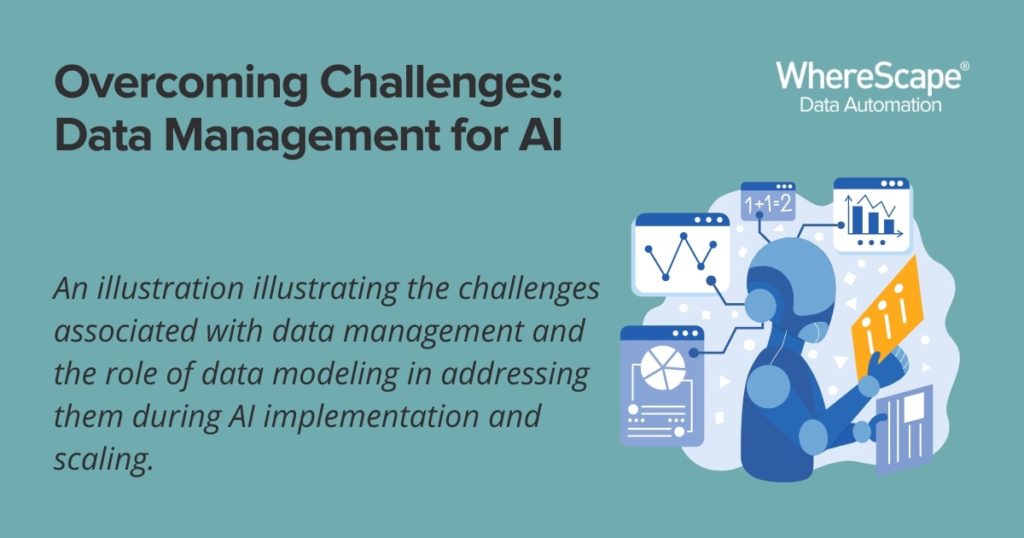
Challenges Faced While Implementing and Scaling AI
Implementing and scaling AI in industries comes with a unique set of challenges, many of which revolve around data management. One of the primary challenges is ensuring data quality, as AI systems heavily rely on large volumes of accurate, up-to-date, and consistent data. Maintaining data privacy is another critical issue, especially when dealing with sensitive information in sectors like healthcare and finance.
The accessibility of data can also pose a problem, as data often exists in isolated silos within organizations, hindering its effective use in AI projects. The sheer volume of data needed for effective AI and the lack of standardization across different systems further complicates matters.
Additionally, preparing data for AI, such as labeling and cleaning it, can be time-consuming and expensive. Another concern is the potential for AI to reproduce bias from the training data, leading to potentially unfair outcomes.
Finally, the lack of skills and expertise in managing big data and AI, the complexities of data governance, and the difficulty of shifting an organization’s culture to become more data-driven also present substantial hurdles. These challenges require strategic, technological, and talent development solutions to implement and scale AI successfully.
Common Problems Across Sectors Related to Data Management
To recap, while AI has undoubtedly transformed many industries, implementing and scaling it isn’t without challenges. Here’s a quick list of some of the common problems across sectors related to data management for AI include:
- Data Quality: AI systems rely on large quantities of data for training and operation. If the data is correct, complete, updated, and consistent, it can lead to accurate outcomes and predictions.
- Data Privacy: Protecting data privacy and security remains a critical challenge, especially in industries like healthcare and banking, where sensitive personal information requires strict safeguards.
- Data Accessibility: Different departments often store data in silos, making it difficult to access and use effectively for AI projects.There might also be restrictions and regulations governing data access.
- Data Volume: Managing the sheer data required for effective AI can be challenging. Storing, processing, and analyzing massive amounts of data requires robust infrastructure and resources.
- Data Labeling and Preparation: AI often requires data to be labeled or annotated, especially in supervised learning scenarios. This can be a time-consuming and costly process. Cleaning and preparing data for use in AI systems is a significant hurdle.
- Lack of Standardization: There might be inconsistencies in how data is collected and recorded across different systems within an industry or the same organization. This lack of standardization can make it challenging to aggregate and analyze the data.
- Bias in Data: AI systems can learn and reproduce bias in the training data, leading to unfair or discriminatory outcomes. This is a particular concern in sectors like HR and law enforcement.
- Data Governance: Setting clear policies for data governance is a complex but essential task, particularly in large organizations or heavily regulated industries.
- Lack of Skills and Expertise: Many organizations need help finding professionals with the right skills to work with big data and AI. This skill gap can slow down AI implementation and scaling.
- Change Management: Shifting an organization’s processes and culture to become data-driven and AI-ready can be a significant challenge.
Organizations can overcome these substantial hurdles in AI implementation and scaling through strategy, technology, and talent development.
Data modeling is the foundation of AI data management, defining how data is structured, stored, and utilized within an AI system. A well-designed data model enables:
- High-quality data ingestion – Ensuring AI systems receive clean, well-structured data.
- Efficient data processing – Reducing redundancy and improving data retrieval speed.
- Interoperability – Facilitating seamless integration between AI models and diverse data sources.
- Scalability – Allowing AI models to adapt to growing datasets without performance degradation.
Modern data modeling tools, such as WhereScape, automate and streamline these processes, ensuring that businesses can implement AI with minimal friction.
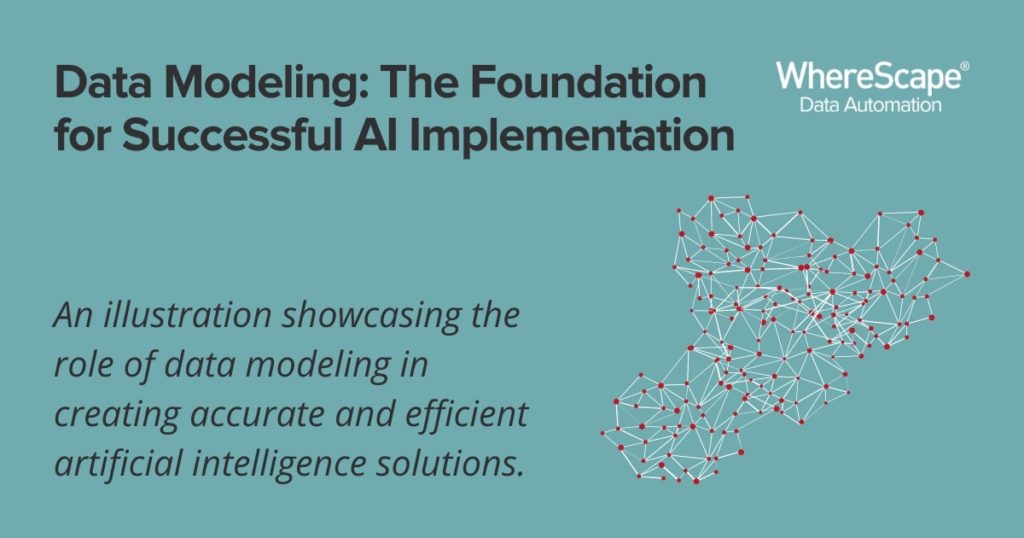
How Data Warehouse Automation Enhances AI Data Management
Data Warehouse Automation (DWA) transforms AI data management by providing a structured and efficient approach, providing a structured and efficient approach to handling large volumes of data. With AI-dependent applications requiring vast amounts of high-quality data, DWA ensures that data is clean, well-structured, and ready for AI processing. Here’s how:
- Improved Data Reliability: By automating the ingestion, transformation, and integration of data, DWA significantly reduces human error, ensuring data accuracy and consistency.
- Faster Data Preparation: AI models need well-prepared, structured data to function optimally. DWA automates data cleaning, reducing the time and effort required for AI-ready datasets.
- Enhanced Scalability: As AI workloads grow, organizations need scalable data pipelines. DWA solutions automate the scaling of data management processes, allowing AI applications to handle increasing volumes of data effortlessly.
- Better Data Governance and Compliance: DWA enforces data lineage tracking, governance, and security policies, making it easier for organizations to comply with data protection regulations while maintaining AI transparency.
- Seamless Data Integration: Multiple data sources feed into AI applications, requiring seamless integration. DWA facilitates real-time data integration, enabling AI models to access updated, relevant data for accurate decision-making.
By incorporating DWA into AI strategies, businesses can maximize the efficiency and reliability of their AI models, leading to more accurate predictions, improved decision-making, and faster insights.
Data Model Automation
The Solution: Data Modeling with Modern Tools Like WhereScape
Data modeling, especially with modern automation tools like WhereScape, can address many challenges associated with working with data for AI implementation and scaling. Here’s how:
- Data Quality: Data modeling ensures data is correctly structured and consistent, improving its quality. It provides a blueprint that guides data storage, management, and use, reducing the likelihood of errors and inconsistencies. Tools like WhereScape automate the data modeling process, further ensuring quality and accuracy.
- Data Accessibility: A good data model can break down data silos, making data more easily accessible for AI projects. Defining transparent relationships between elements enables more effective data integration across the organization. Automation tools can expedite this process, making accessing and using data for AI quicker.
- Data Volume: Modern data modeling tools help manage large volumes of data by enabling more efficient data architectures. They can automate the design, development, and deployment of data infrastructure, making handling the significant datasets standard in AI easier.
- Data Labeling and Preparation: Data modeling guides the data preparation process by defining what data to include in the model and how to format and label it. Automation can speed up this process, reducing the time it takes to prepare data for AI.
- Lack of Standardization: Data modeling introduces standards for data structure and management. This standardization makes aggregating and analyzing data, even from different systems or departments, more accessible.
- Data Governance: Data modeling supports governance by providing a clear framework for data management. It helps define who can access data, what they can do with it, and how it should be stored and protected. Tools like WhereScape can help enforce these policies by automating the data management process.
- Lack of Skills and Expertise: Professionals without data modeling expertise can use modern data modeling tools. These tools provide user-friendly interfaces and features that guide users through the modeling process, making it accessible to a broader range of professionals.
- Change Management: A transparent data model helps organizations transition to a more data-driven culture. It clearly represents how data will be used, making it easier for stakeholders to understand and support the shift. Automation streamlines this process by improving data management efficiency and reducing errors.
While data modeling doesn’t solve every challenge—such as data privacy, data bias, and certain aspects of change management—it plays a crucial role in managing data effectively for AI implementation and scaling.
Key Roles in Business and Triggers for Modern Data Modeling Tools
Several organizational roles might identify the need for modern data modeling tools. Some of these roles, their responsibilities, and their triggers include:
- Chief Data Officers (CDOs) and Data Managers: Responsible for data governance, data privacy, and the overall management of the company’s data. If they notice data silos or data inconsistencies or find it difficult to enforce data policies, it’s a sign they might need a modern data modeling tool.
- Chief Technology Officers (CTOs) and IT Managers: Responsible for managing the technological infrastructure. If they need help with data volume and data integration from different systems, or if the current infrastructure cannot support the advanced data needs of AI, they should consider switching to modern data modeling tools.
- Data Scientists and Data Analysts: These roles involve working directly with data to develop AI models and draw insights. A modern data modeling tool could improve efficiency if they spend too much time cleaning, preparing, and managing data or need help standardizing data from different sources.
- Chief Information Officers (CIOs): Responsible for information technology strategy. If they see a need for more standardization across data sources or if the organization’s data strategy does not align with its AI goals, they may suggest adopting advanced data modeling tools.
- Business Intelligence (BI) Leaders: They oversee the transformation of data into actionable business insights. If they cannot access the data they need, or if data quality affects the accuracy of senses, it may be time to consider a modern data modeling tool.
- Project Managers and Product Managers: If data-related issues delay AI solution development and implementation or if they need help coordinating teams working with data, modern data modeling tools can help.
- AI Specialists: They are responsible for designing, training, and implementing AI models. If they cannot access high-quality, relevant data for training AI models, they might see the need for modern data modeling tools.
- Data Stewards: They manage and maintain data assets to ensure reliable, high-quality data. It could indicate the need for modern data modeling tools if they need help with data quality, consistency, or metadata management.
The WhereScape Advantage in AI-Driven Data Management
WhereScape provides an advanced Data Warehouse Automation platform that simplifies and accelerates AI data management. It enhances AI applications by automating the full data lifecycle—from ingestion to modeling and deployment—ensuring businesses get the most reliable and high-quality data for AI processing.
WhereScape 3D: Automating Data Modeling for AI Success
WhereScape 3D is a powerful data modeling tool that enables organizations to design and prototype data architectures efficiently. It plays a critical role in AI data management by:
- Automating Data Discovery: WhereScape 3D rapidly profiles and visualizes data structures, helping organizations understand their data assets before implementing AI solutions.
- Accelerating Data Modeling: Automates the creation of conceptual, logical, and physical data models, reducing manual effort and expediting AI development.
- Improving Data Quality: Ensures AI applications receive structured, validated, and optimized data models, reducing inconsistencies and inaccuracies.
- Facilitating Collaboration: Provides a metadata-driven framework that aligns data engineers, data scientists, and business users for streamlined workflows.
- Enhancing Data Governance: Supports AI-ready governance policies by enforcing data consistency, lineage tracking, and compliance with industry regulations.
WhereScape 3D streamlines the modeling process, ensuring that AI teams can quickly develop and refine data architectures that support AI workloads effectively.
WhereScape RED: End-to-End Data Automation for AI Workflows
WhereScape RED takes automation a step further by handling the entire data lifecycle, from ingestion to deployment. Key benefits of WhereScape RED for AI data management include:
- Automated Data Pipelines: Simplifies ETL/ELT processes, ensuring AI models receive continuous, up-to-date data.
- Metadata-Driven Development: Tracks data lineage, transformations, and dependencies, enhancing AI interpretability and trust.
- Seamless Integration: Works across cloud, on-premises, and hybrid environments, making AI implementations more flexible.
- Optimized Performance: Reduces manual coding efforts, enabling faster and more efficient AI data processing.
- Built-in Orchestration: Automates scheduling and executes data workflows, ensuring AI models train with the latest data without manual intervention.
- Cross-Platform Compatibility: Supports leading cloud data platforms such as Snowflake, Azure, AWS, Databricks, and Google Cloud, providing organizations with a flexible AI-ready data ecosystem.
By combining WhereScape 3D for data modeling and WhereScape RED for automation, businesses can create an AI-ready data architecture that is scalable, reliable, and optimized for analytics and decision-making.
Why Choose WhereScape for AI Data Management?
- Reduced AI Development Time: Automated workflows eliminate bottlenecks in AI data preparation.
- Improved AI Model Accuracy: Structured and well-governed data leads to better AI predictions.
- Enhanced Compliance and Security: Built-in governance features ensure compliance with global data regulations.
- Optimized AI-Ready Data Pipelines: Ensures seamless data flow, making AI implementations more effective and reliable.
- Scalability and Flexibility: Supports a wide range of data environments, allowing organizations to scale AI workloads without operational friction.
WhereScape empowers businesses to bridge the gap between data management and AI, enabling seamless, automated, and efficient AI-driven analytics. By leveraging WhereScape, organizations can fully unlock the power of AI through structured, high-quality data automation.
AI Success Starts with Data Modeling
As AI adoption accelerates, data modeling will continue to be a critical component of AI data management. Organizations that invest in modern, automated data modeling solutions like WhereScape 3D and WhereScape RED will benefit from improved data governance, higher AI accuracy, and greater scalability.
By leveraging data modeling automation, businesses can overcome the biggest AI challenges—ensuring high-quality, structured data that powers more reliable, ethical, and impactful AI applications.
Ready to optimize your AI strategy? Discover how WhereScape’s automated data modeling tools can streamline AI data management today and Request a demo.
WhereScape at TDWI Munich: Automate Data Vault on Databricks
WhereScape at TDWI Munich 2025: Automate a Full Data Vault on Databricks in Just 45 Minutes June 24–26, 2025 | MOC Munich, Germany As data complexity grows and business demands accelerate, scalable and governed data architectures are no longer optional—they're...
What Is OLAP? Online Analytical Processing for Fast, Multidimensional Analysis
Streamline your data analysis process with OLAP for better business intelligence. Explore the advantages of Online Analytical Processing (OLAP) now! Do you find it challenging to analyze large volumes of data swiftly? A Forrester study reveals that data teams spend...
Build AI-Ready Data: Visit WhereScape at AI & Big Data Expo
June 4–5, 2025 | Booth 202 | Santa Clara Convention Center As organizations scale their artificial intelligence and analytics capabilities, the demand for timely, accurate, governed, and AI-ready data has become a strategic priority. According to Gartner, through...
Automating Star Schemas in Microsoft Fabric: A Webinar Recap
From Data Discovery to Deployment—All in One Workflow According to Gartner, data professionals dedicate more than half of their time, 56%, to operational tasks, leaving only 22% for strategic work that drives innovation. This imbalance is especially apparent when...
What is a Data Model? How Structured Data Drives AI Success
What is a data model? According to the 2020 State of Data Science report by Anaconda, data scientists spend about 45% of their time on data preparation tasks, including cleaning and loading data. Without well-structured data, even the most advanced AI systems can...
ETL vs ELT: What are the Differences?
In working with hundreds of data teams through WhereScape’s automation platform, we’ve seen this debate evolve as businesses modernize their infrastructure. Each method, ETL vs ELT, offers a unique pathway for transferring raw data into a warehouse, where it can be...
Dimensional Modeling for Machine Learning
Kimball’s dimensional modeling continues to play a critical role in machine learning and data science outcomes, as outlined in the Kimball Group’s 10 Essential Rules of Dimensional Modeling, a framework still widely applied in modern data workflows. In a recent...
Automating Data Vault in Databricks | WhereScape Recap
Automating Data Vault in Databricks can reduce time-to-value by up to 70%—and that’s why we hosted a recent WhereScape webinar to show exactly how. At WhereScape, modern data teams shouldn't have to choose between agility and governance. That's why we hosted a live...
WhereScape Recap: Highlights From Big Data & AI World London 2025
Big Data & AI World London 2025 brought together thousands of data and AI professionals at ExCeL London—and WhereScape was right in the middle of the action. With automation taking center stage across the industry, it was no surprise that our booth and sessions...
Why WhereScape is the Leading Solution for Healthcare Data Automation
Optimizing Healthcare Data Management with Automation Healthcare organizations manage vast amounts of medical data across EHR systems, billing platforms, clinical research, and operational analytics. However, healthcare data integration remains a challenge due to...
Related Content
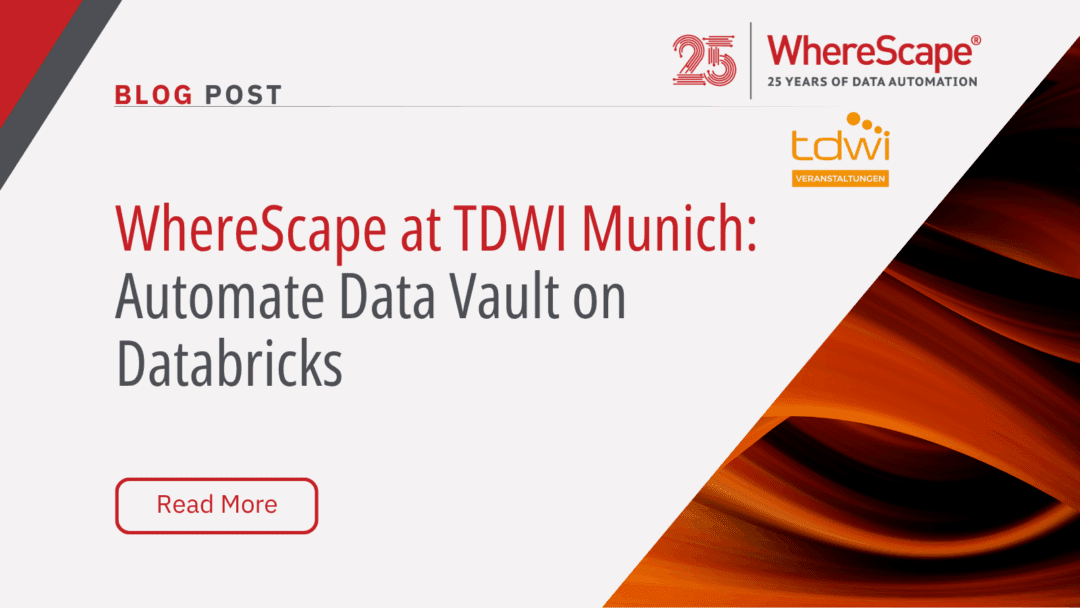
WhereScape at TDWI Munich: Automate Data Vault on Databricks
WhereScape at TDWI Munich 2025: Automate a Full Data Vault on Databricks in Just 45 Minutes June 24–26, 2025 | MOC Munich, Germany As data complexity grows and business demands accelerate, scalable and governed data architectures are no longer optional—they're...
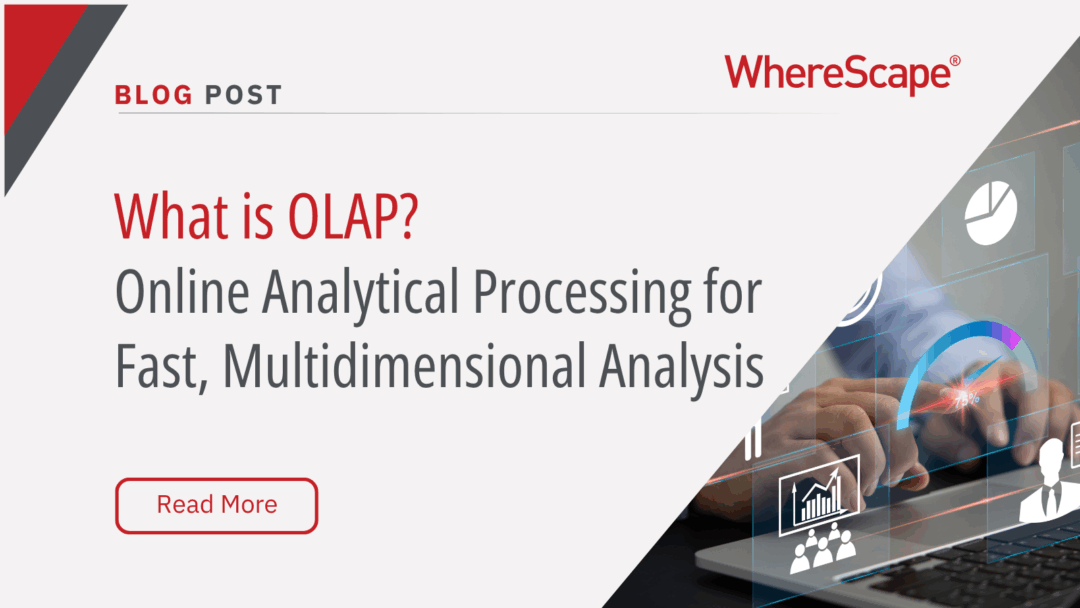
What Is OLAP? Online Analytical Processing for Fast, Multidimensional Analysis
Streamline your data analysis process with OLAP for better business intelligence. Explore the advantages of Online Analytical Processing (OLAP) now! Do you find it challenging to analyze large volumes of data swiftly? A Forrester study reveals that data teams spend...
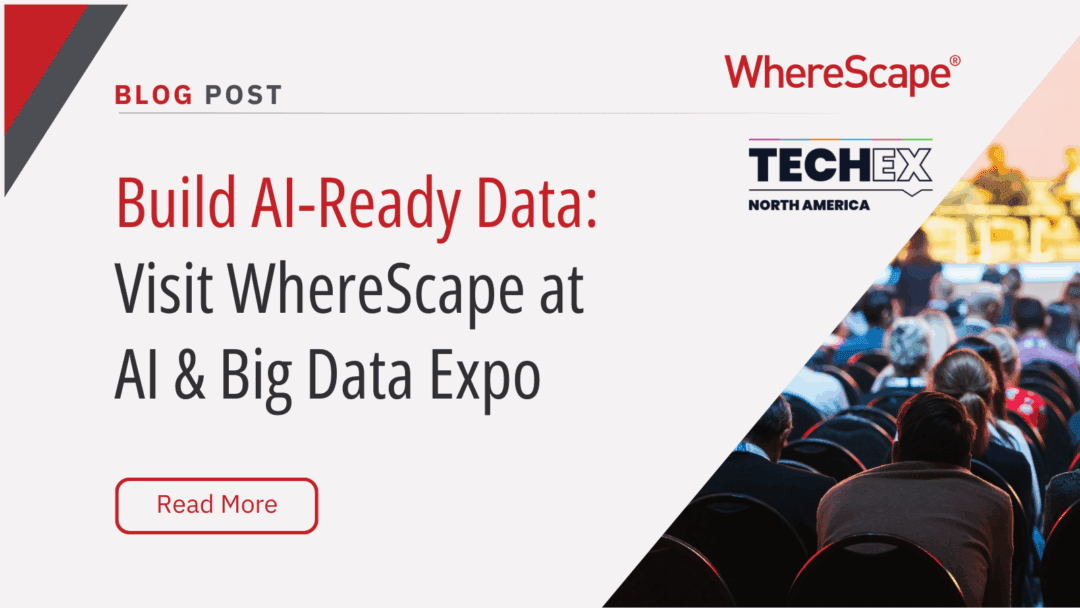
Build AI-Ready Data: Visit WhereScape at AI & Big Data Expo
June 4–5, 2025 | Booth 202 | Santa Clara Convention Center As organizations scale their artificial intelligence and analytics capabilities, the demand for timely, accurate, governed, and AI-ready data has become a strategic priority. According to Gartner, through...
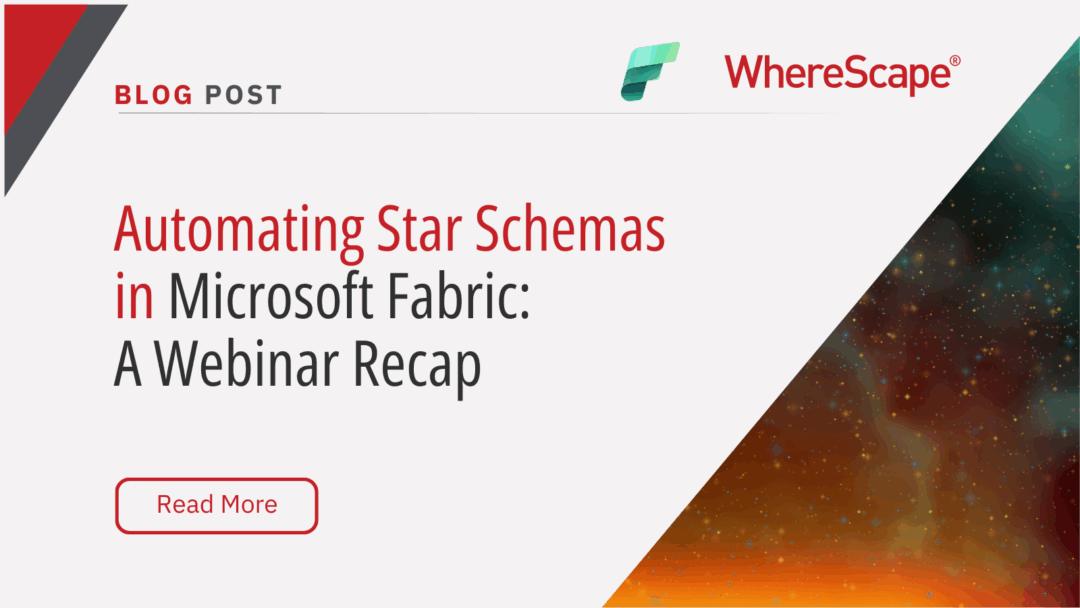
Automating Star Schemas in Microsoft Fabric: A Webinar Recap
From Data Discovery to Deployment—All in One Workflow According to Gartner, data professionals dedicate more than half of their time, 56%, to operational tasks, leaving only 22% for strategic work that drives innovation. This imbalance is especially apparent when...