Are you struggling to keep up with the...
8 Reasons to Make the Switch to ELT Automation
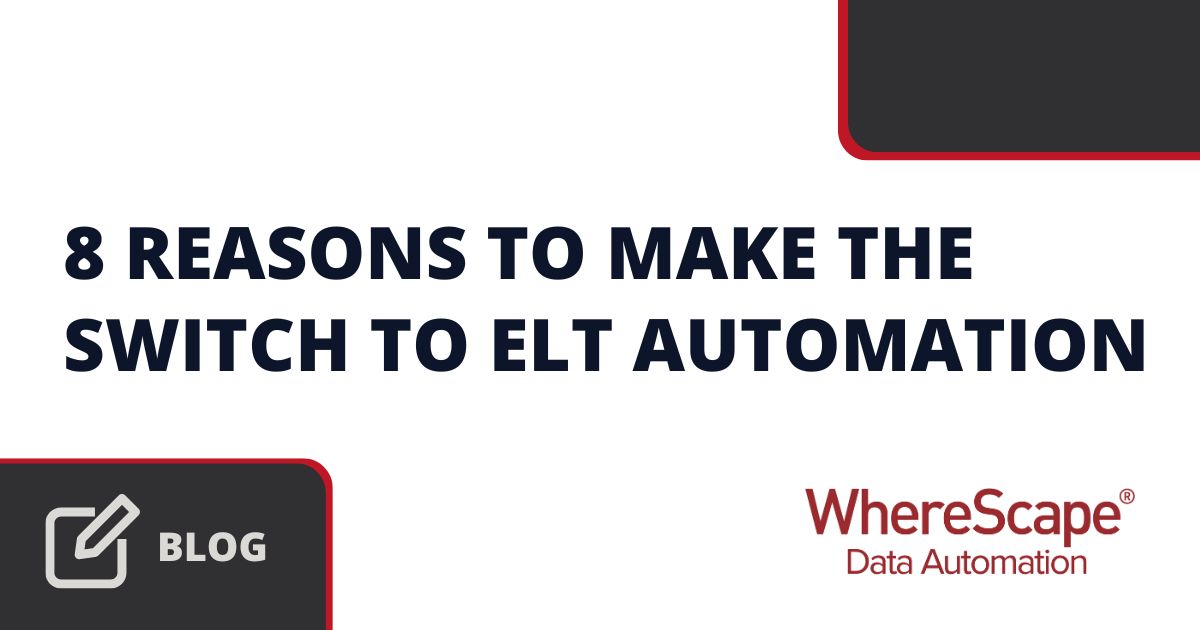
Extraction, loading, and transformation (ELT) processes have been in existence for almost 30 years. It has been a programming skill set mandatory for those responsible for the creation of analytical environments and their maintenance because ELT automation works. Sadly though, ELT alone is not good enough to keep up with the speed at which modern analytical needs change and grow.
ELT Process
The increasingly complex infrastructures of most analytical environments, the addition of massive amounts of data from unusual sources, and the complexity of the analytical workflows all contribute to the difficulties that implementation teams have in meeting the needs of the business community. Just the length of time it takes to create a new report – a relatively simple process – demonstrates that just having traditional ELT skills is not enough. We must improve and speed up all data integration by introducing automation into ELT processes.
Automating is more than just relieving the implementers of creating over and over the many mundane and repetitive tasks. Among its many benefits are the following:
1. Automated Documentation
Automation ensures that the ELT processes are not just tracked but documented in terms of up-to-date metadata on every extraction, every transformation, every movement of the data, and every manipulation performed on it as it makes its way to the ultimate analytical asset (a report, an analytic result, a visualization, a dashboard widget, and so on).
This metadata is not an afterthought; it is integral to the automation software itself and is always current. It is as useful to the business community as it is to the technical implementation staff. Business users increase their adoption of analytical assets if they can determine that the asset was created from the same data they would have used, that it was properly integrated with other sets of data and that the ultimate analytical asset is exactly what they need. In other words, they trust the data and assets.
2. Document Process Automation
By setting up routine programs to handle common tasks like date and time processing, reference and look-up tables, and serial key creation, the analytical teams establish much-needed standards. The implementers can spin up new data and analytical assets or perform maintenance on existing assets without introducing “creative” (non-standard) data into these critical components. No matter where the data resides (on-premises, in the cloud, in a relational database, or not), these sets of data remain the same, making their utilization so much easier by all (business community or technical staff).
3. Data Lineage
A significant automation boon to any analytical environment is its automatic creation of the data’s lineage. Data lineage consists of the metadata that shows all the manipulations occurring to data from its source(s) to its ultimate target database as well as the individual operations to produce analytical assets (algorithms, calculations, etc.). Think how useful that information becomes to business users, data scientists, and others using and creating analytical assets. Being able to understand how upstream ELT changes can affect downstream analytical assets eliminates so many problems for users and implementers alike.
4. Faster Time-to-Value
Project lead time is greatly reduced with automation when adopting a new technological target (e.g., moving to Snowflake or Azure Synapse or Databricks) or migrating from an on-premises environment to a cloud-based one. Much of the ELT code generated from automation technology can be easily retrofitted to the new environment through simple pull-down menu options. Minimal additional recoding efforts will be needed. In essence, by adopting automation, an organization is basically “future-proofing” its analytical architecture – no small accomplishment!
5. Agile Methodology
ELT automation supports the technical staff as they move to adopt a more iterative and agile methodology. Rather than having a series of discrete steps in a traditional methodology with hand-offs between staff, all the steps for data integration are encapsulated in the automation tool so that moving from one step to another is seamless and fast. The same resource can perform all the data integration steps without any handoffs. This makes the adoption of an agile methodology not only possible but compelling.
6. Data Governance
By capturing all the technical metadata and ensuring its accuracy and currency, automated ELT serves another audience nicely – the data governance function. Understanding the full life cycle of data integration from initial capture to ultimate target, data stewards can monitor where the data came from (approved sources or not), what changes and transformations were performed on it (standard calculations or personalized ones), and what analytical assets can now be certified (“Enterprise-approved” or “Corporate Standards”).
7. Data Modeling
One of the more difficult migrations an analytical environment may go through is a change in its data modeling style. For example, switching from a star schema-based data warehouse to one based on the Data Vault design. Without data integration automation and well-documented metadata, this change would require a total rewriting of all ELT code.
With automation, all the steps leading to the ultimate storage of the data may be preserved and only the last few processes that create the database schema and load the data would have to be altered. Much of the intellectual capital can be preserved and the change made quickly and efficiently.
8. Data Fabric
Finally, many organizations are considering a new architecture to replace their aging data warehouses – the “Data Fabric”. The idea of a data fabric started in the early 2010s. Since then, many papers, vendors, and analyst firms have adopted the term. The goal of a data fabric is to create an architecture that encompasses all forms of analytical data for any type of analysis (e.g., from straightforward reporting to complex business analysis to complicated data science explorations) with seamless accessibility and shareability by all those with a need for it.
Data in a data fabric may be stored anywhere throughout the enterprise which makes automated ELT a mandatory tool for increasing the likelihood of success in this new endeavor. Well-documented ELT greatly reduces the overall complexity by streamlining the creation and maintenance of this highly distributed environment.
The Strategic Advantage of ELT Automation
ELT Automation transcends mere data handling improvements; it’s a strategic revolution in data integration. This transformation brings critical advantages that are essential in the labyrinth of today’s analytical landscapes. Not only does it cater to the technical workforce by streamlining operations and reducing errors, but it also aligns with business objectives by enabling agility and ensuring data integrity.
In an era where traditional, cumbersome ELT methodologies falter under the weight of complexity and speed, ELT Automation emerges as the linchpin for enterprises aiming to swiftly adapt and innovate. By embracing automation, organizations are not just enhancing their data processes—they are securing a competitive edge, ensuring that they can swiftly respond to market changes and seize new opportunities without compromising on the quality or accuracy of their analytical assets. It’s not just about keeping up; it’s about setting the pace.
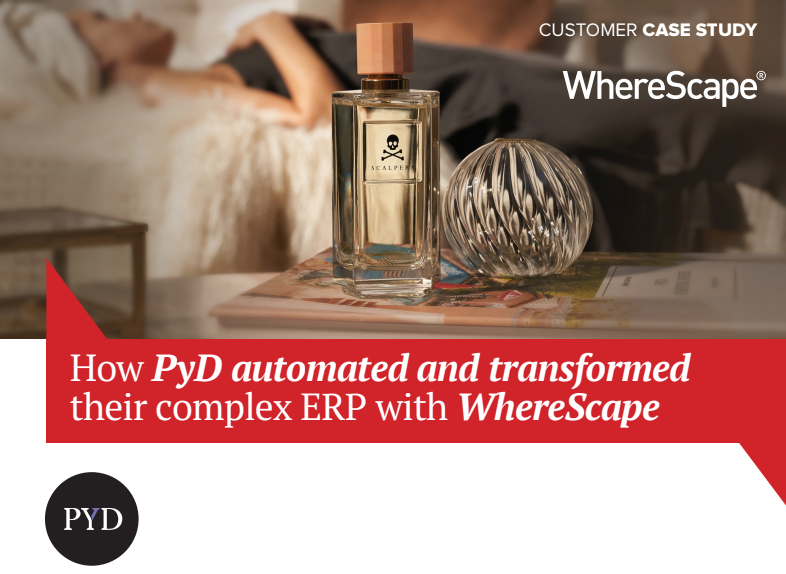
Automating ELT: The PyD and WhereScape Success Story
PyD, a luxury perfume and cosmetics company based in Madrid, Spain, faced a significant challenge with its complex ERP system. With 220 employees and a global presence, PyD needed to streamline its data processing for Business Intelligence purposes, overcoming inefficiencies and manual error risks inherent in its existing setup.
The Challenge
The primary obstacle for PyD was the cumbersome, non-standardized ERP system that made data management and report generation time-consuming and prone to errors. This complexity hindered the company’s ability to develop efficient data warehouse structures and processes.
The Solution
PyD turned to WhereScape, a leading data automation solution provider, implementing WhereScape® 3D and WhereScape® RED. These tools were chosen not just for their ETL capabilities but for their comprehensive approach to designing, developing, deploying, and operating the entire data warehouse.
The Results
With WhereScape, PyD was able to automate the design and development of ELT routines for its ERP system, significantly improving data availability and format accuracy for analysis. This led to a unified reporting system, streamlined processes, and future-proofed data management practices. Iván San José, PyD’s IT Director, highlighted the software’s ease of use and versatility in solving complex data challenges, noting its potential for future competitiveness.
PyD’s experience illustrates the transformative potential of ELT automation in modern data management. By adopting WhereScape’s solutions, PyD not only addressed its immediate challenges but also positioned itself for ongoing success in a rapidly evolving business landscape. This case study demonstrates the importance of integrating automation into ELT processes to enhance efficiency, reliability, and strategic adaptability. Read the full case study here.
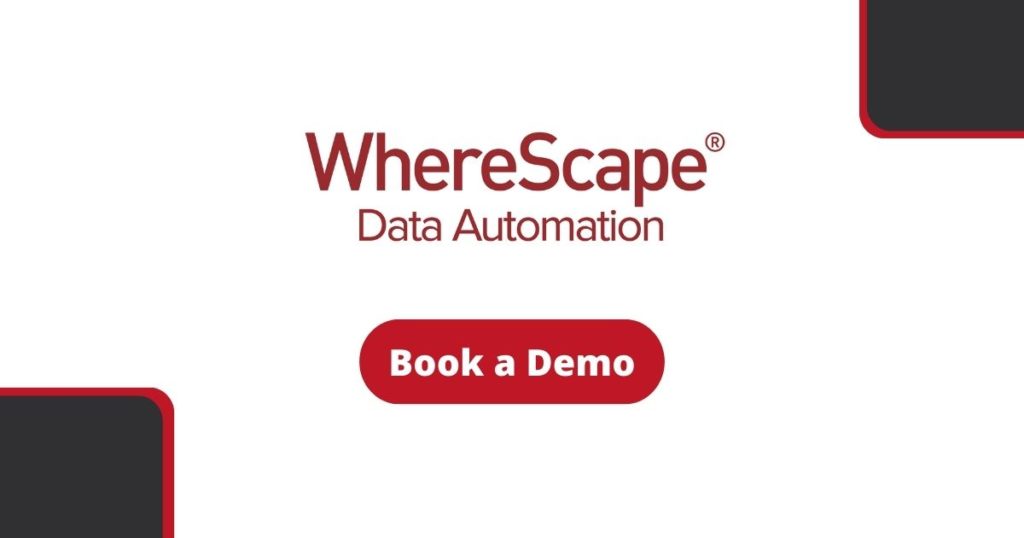
Elevate Your Data Strategy with ELT Automation
The digital era demands swift, reliable data management, and ELT Automation stands out as the strategic solution. We’ve outlined the undeniable advantages of integrating ELT Automation into your data processes, highlighting its role in streamlining operations, enhancing data governance, and accelerating project timelines. Through PyD’s success story, we showcased the profound impact of WhereScape’s automation solutions on business intelligence and data management efficiency.
Embrace the change. ELT Automation is your gateway to a future-proof data ecosystem, offering unmatched operational efficiency and strategic agility. Book a demo with WhereScape today, and take the first step towards transforming your data management processes. It’s time to lead with confidence in a data-driven world.
A Webinar Recap: Exploring Data Automation Levels with Kent Graziano
Our most recent webinar, "The Future of Data Warehousing: Understanding Automation Levels," hosted by Patrick O'Halloran, Solutions Architect, and esteemed guest speaker Kent Graziano dove into the transformative world of data warehouse automation. They discussed its...
WhereScape’s Supported Platforms: Accelerating Data Solutions Across the Board
The Future of Data Warehouse Automation with WhereScape Data warehouse automation represents a transformative shift in how businesses manage and utilize their data. WhereScape is at the forefront of this movement, offering tools that automate code generation,...
Overcoming Challenges with AI Hallucinations
Conversing with your digital assistant on your smartphone, using facial recognition for security, traveling in autonomous vehicles, or browsing recommended products based on your search history - there is no denying AI is embedded in many aspects of our lives. AI has...
Navigating Data Governance with WhereScape 3D
Properly managing and organizing data allows businesses to not only understand crucial patterns and trends, but also to leverage that data in strategic ways that grow revenue over time. Data drives decision-making and paves the way for innovation when used properly....
Deep Dive into WhereScape RED: Features and Benefits
Transforming a business’s various databases and files into actionable insights and reports is crucial, but incredibly time-consuming with traditional tools. Fortunately, with data warehouse automation tools like WhereScape RED, organizations can take advantage of a...
Brief Insights from Gartner® Latest Report on Data Fabric and Data Mesh
In the rapidly evolving world of data management, distinguishing between the myriad of strategies and technologies can be daunting. The latest Gartner® report, "How Are Organizations Overcoming Issues to Start Their Data Fabric or Mesh?" provides critical insights...
ETL vs ELT: What are the Differences?
In data management, the debate between ETL and ELT strategies is at the forefront for organizations aiming to refine their approach to handling vast amounts of data. Each method, ETL vs ELT, offers a unique pathway for transferring raw data into a warehouse, where it...
Embracing the Future of Data Management Recap: Insights from Mike Ferguson
In our recent webinar, "Embrace the Future of Data Management with Automated Cloud Data Warehousing," we had the privilege of diving into the transformative world of cloud data warehousing and highlighting the pivotal role of automation. Guided by our own Brad Kloth,...
How to Hire and Retain Data Warehouse Developers
The projected data warehouse developer job growth rate is 21% from 2018-2028, with about 284,100 new jobs for data warehouse developers projected over the next decade, according to Zippia. This surge in demand for data warehouse talent is being felt across businesses...
Empowering Data Excellence: WhereScape Joins Forces with Peloton Data Solutions
WhereScape is thrilled to announce an exciting new partnership with Peloton Data Solutions, a beacon of data warehouse expertise in New Zealand. This collaboration unites two leaders in data management and automation, poised to set new standards in data warehouse...
Related Content
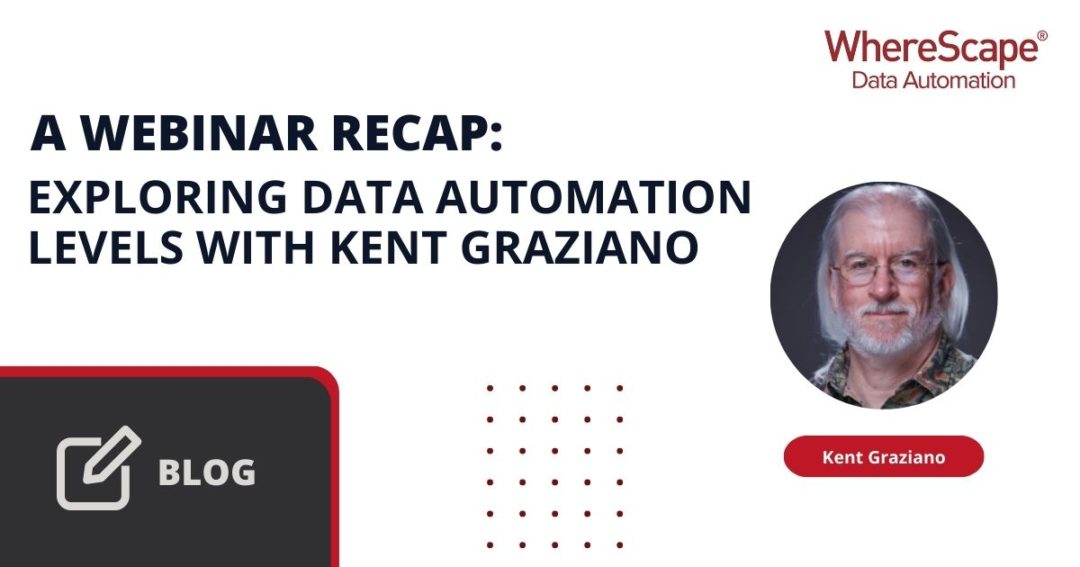
A Webinar Recap: Exploring Data Automation Levels with Kent Graziano
Our most recent webinar, "The Future of Data Warehousing: Understanding Automation Levels," hosted by Patrick O'Halloran, Solutions Architect, and esteemed guest speaker Kent Graziano dove into the transformative world of data warehouse automation. They discussed its...
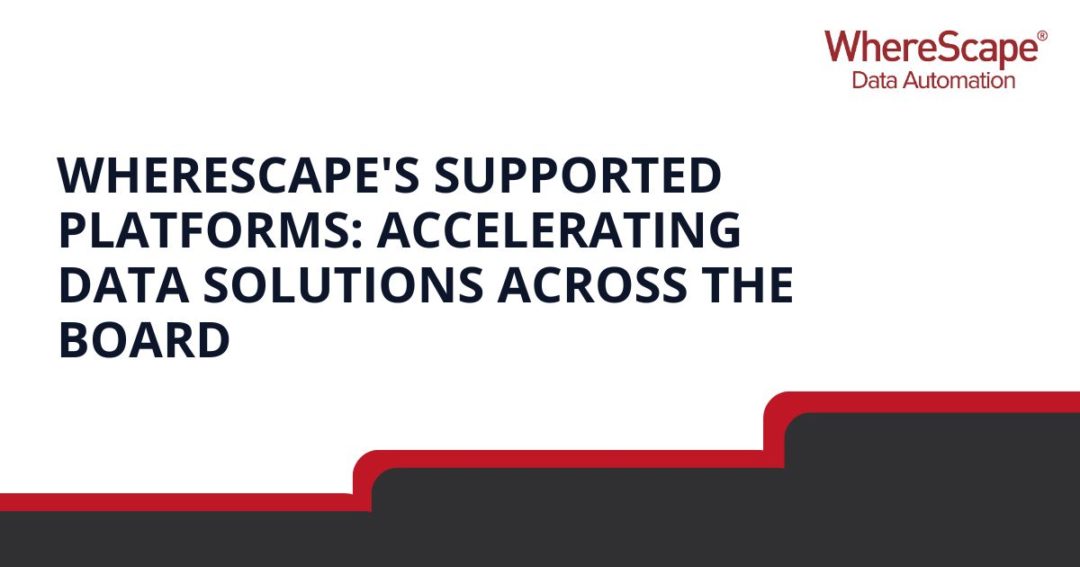
WhereScape’s Supported Platforms: Accelerating Data Solutions Across the Board
The Future of Data Warehouse Automation with WhereScape Data warehouse automation represents a transformative shift in how businesses manage and utilize their data. WhereScape is at the forefront of this movement, offering tools that automate code generation,...
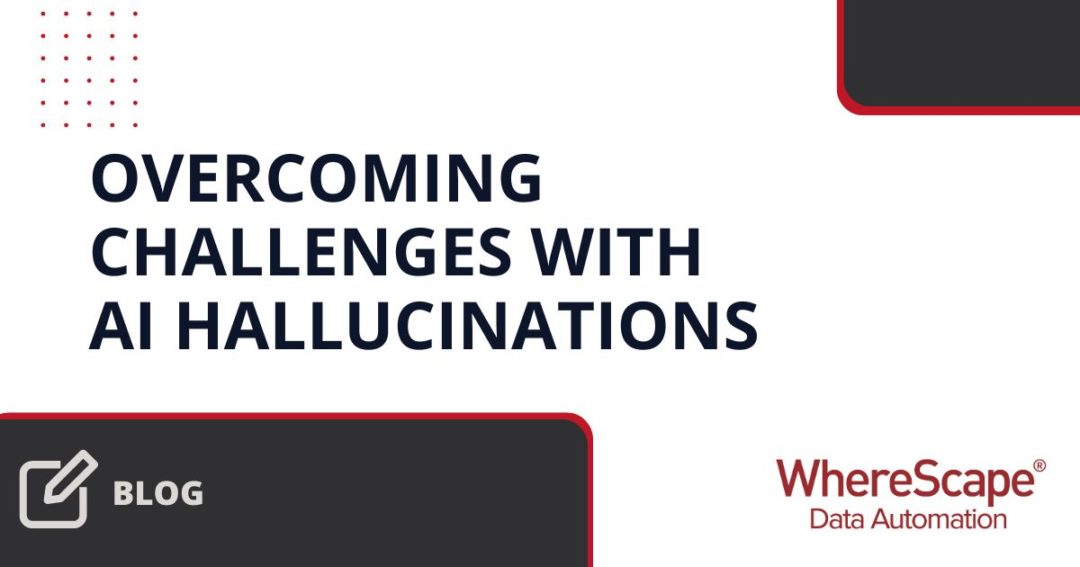
Overcoming Challenges with AI Hallucinations
Conversing with your digital assistant on your smartphone, using facial recognition for security, traveling in autonomous vehicles, or browsing recommended products based on your search history - there is no denying AI is embedded in many aspects of our lives. AI has...
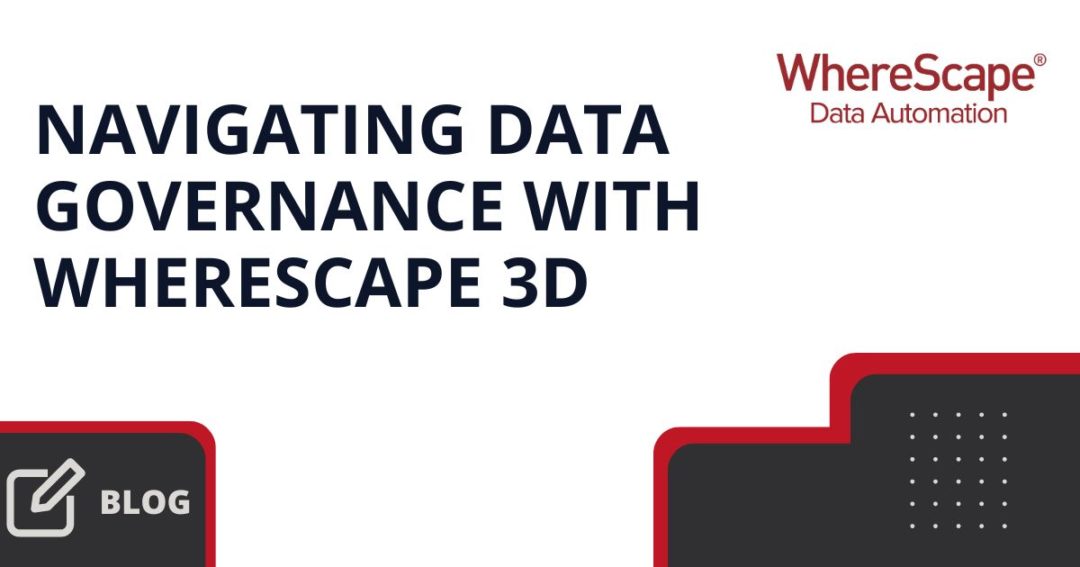
Navigating Data Governance with WhereScape 3D
Properly managing and organizing data allows businesses to not only understand crucial patterns and trends, but also to leverage that data in strategic ways that grow revenue over time. Data drives decision-making and paves the way for innovation when used properly....