Maximize Value, Minimize Expenses.
WhereScape RED
RED is the only tool you need to ingest, transform, build, deploy and manage
your data solution.
WhereScape 3D
3D is a GUI-based data solution designer that helps you quickly build and deploy your data model.
WhereScape DVE
Data Vault Express is a specialized tool to automate building Data Vault solutions faster and more efficiently.
The Results.
Organizations who use WhereScape Data Automation Report:
95% TIME-SAVINGS
on hand-coding development, refactoring and management tasks.
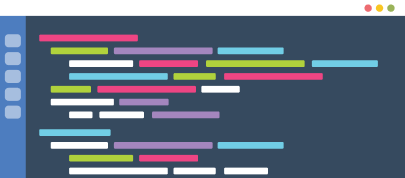
8x DEVELOPER PRODUCTIVITY
in implementing and managing infrastructure through automation.
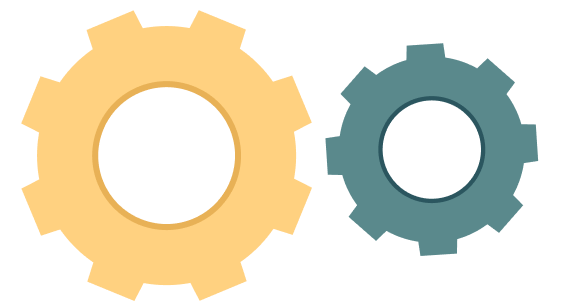
6x RETURN ON INVESTMENT
by avoiding failures, filling skill gaps and adding built-in best practices.
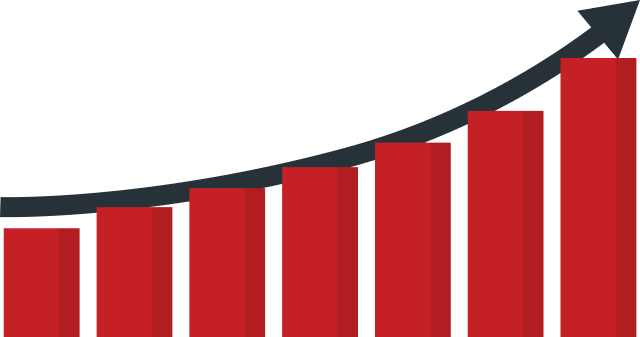
WhereScape is the One Constant You Can Rely On
WhereScape integrates seamlessly with leading data platforms, including Snowflake, Databricks, SQL Server, and more to provide you with ultimate flexbility.
WhereScape + Snowflake
Get more value from your Snowflake investment sooner with WhereScape. WhereScape for Snowflake automates workflows based on native Snowflake functions, wizards, and best practices, reducing time to delivery and costs.
WhereScape + Databricks
Automate data ingestion, machine learning lifecycles, end-to-end data pipeline development, and more in your Databricks workspace with WhereScape. Achieving efficient data management has never been easier.
WhereScape + Azure Synapse
Accelerate your Azure data warehouse and deliver near-real-time data to the business with WhereScape’s built-in design patterns, configurable templates, automatically generated SQL-based ETL code, and more.
Thousands of Customers Trust WhereScape
Modernize Your Data Warehouse
Increase Developer Productivity and Reduce Risk.
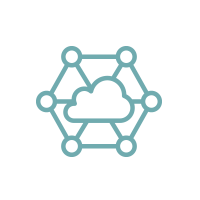
Cloud Data Warehouse Automation
Harness the cloud for all or part of your data infrastructure to gain more and pay less.
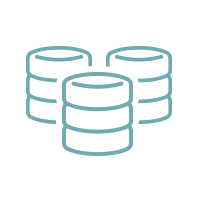
Big Data
Integrate big data with other enterprise data sources for comprehensive insights.
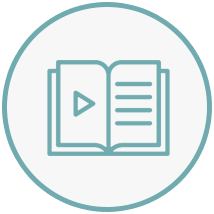
Documentation
Use metadata to automatically produce full documentation, including lineage.
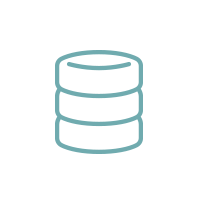
Data Warehouse
Empower your organization to be data driven with a central source for extracting insights.
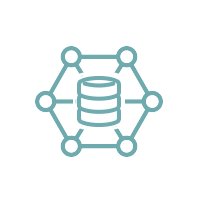
Data Mart
Give users the specific subject areas they need and deliver them quickly with automation.
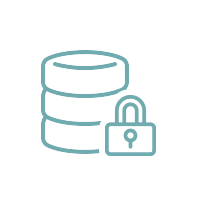
Data Vault
Easily deliver 2.0 enabled data vaults for greater adaptability to change and growth.
Upcoming WhereScape Events
Latest WhereScape Updates
A Webinar Recap: Exploring Data Automation Levels with Kent Graziano
Our most recent webinar, "The Future of Data Warehousing: Understanding Automation Levels," hosted by Patrick O'Halloran, Solutions Architect, and esteemed guest speaker Kent Graziano dove into the transformative world of data warehouse automation. They discussed its...
WhereScape’s Supported Platforms: Accelerating Data Solutions Across the Board
The Future of Data Warehouse Automation with WhereScape Data warehouse automation represents a transformative shift in how businesses manage and utilize their data. WhereScape is at the forefront of this movement, offering tools that automate code generation,...
Overcoming Challenges with AI Hallucinations
Conversing with your digital assistant on your smartphone, using facial recognition for security, traveling in autonomous vehicles, or browsing recommended products based on your search history - there is no denying AI is embedded in many aspects of our lives. AI has...
Join Our Global Community
of Data Enthusiasts
Join our email list and receive the latest case studies, event updates, product news, and much more.
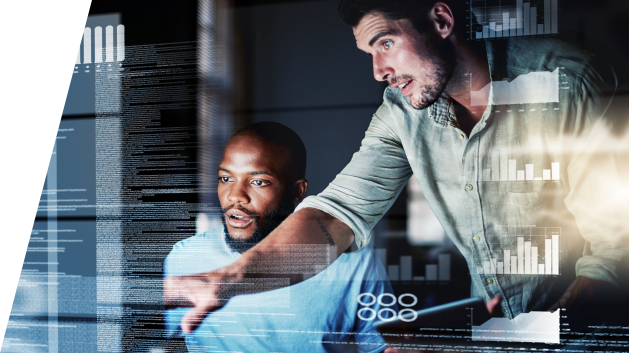