Join us for a 90-minute, insightful webinar...
Effective AI through Data Modeling
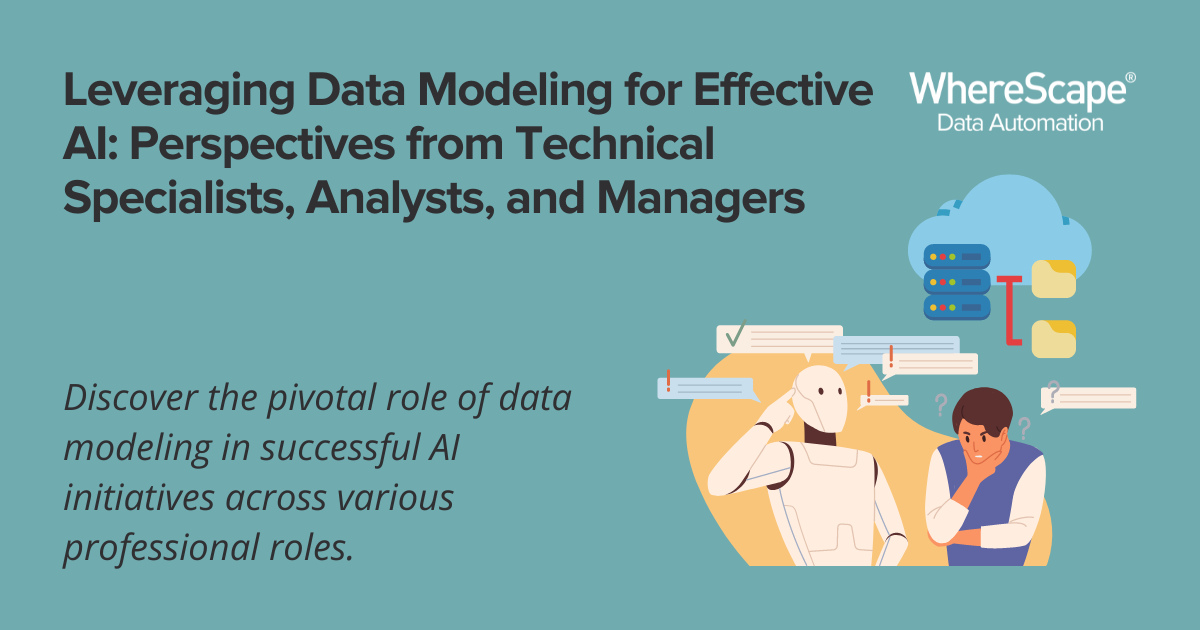
As we journey deeper into the digital age, the importance of data modeling within the broader landscape of artificial intelligence (AI) has become more pronounced than ever. The success of AI-driven initiatives is tightly woven with the quality and structure of the underlying data.
This blog post takes you through the crucial aspect of data modeling, illustrating its importance from the lenses of three key stakeholders: technical specialists, data analysts, and managers.
Importance of Data Modeling
Brief Overview of the Importance of Data Modeling in AI
In the realm of AI, data modeling serves as a structured roadmap for data – a blueprint that is pivotal for the success of AI systems. Data modeling directly influences how effectively data can be utilized, impacting AI initiatives’ speed, efficiency, and accuracy. It is critical for technical specialists tasked with model implementation, data analysts interpreting data, and managers supervising the overall data workflow.
Technical Specialists
Streamlining Work with Models
For technical specialists, the significance of data modeling is multi-dimensional. A robust data model ensures data is accurately organized and readily accessible, significantly streamlining their work. Efficient data modeling reduces time spent on troubleshooting, boosts productivity, and enhances output quality. Using modern automation tools like WhereScape can automate the data modeling process, making creating and maintaining data models more accurate and efficient.
Data Analysts
Enhancing Data Interpretation and Decision Making
Effective data modeling is crucial for data analysts, who transform data into actionable insights. A well-structured data model improves data clarity, consistency, and quality, enabling analysts to generate more precise insights. It drives data-driven decision-making by serving as a reliable platform for extracting meaningful patterns and trends. Tools like WhereScape allow analysts to access and analyze data in a standardized, organized format, making their work more efficient and insightful.
Technical Managers
Accelerating and Optimizing Data Work
Managers who strategize and supervise data work greatly benefit from effective data modeling. It serves as a strategic tool for accelerating and enhancing data workflows. By eliminating data silos and improving data accessibility across departments, a well-defined data model fosters a more data-driven culture within the organization. Automating and streamlining data management processes via tools like WhereScape can lead to faster project completion and more effective resource utilization.
Types of Data Models
Conceptual Data Models
These high-level models provide an overview of the organizational data landscape without detailing specific attributes. Conceptual models are pivotal during the early stages of AI projects, helping identify relationships between different data entities. Utilizing these models can ensure a solid foundation and enhance the overall strategic direction of an AI initiative.
Logical Data Models
Going deeper into data intricacies, logical data models provide a more detailed view of data structures and relationships without focusing on the technical aspects of data storage or retrieval. These models contribute to a more efficient data organization, improving the performance of AI systems by defining more precise data relationships.
Physical Data Models
Physical data models translate logical data into detailed schemas that guide the creation of the database. The effectiveness of physical data models directly affects AI outcomes, as they dictate the specifics of data storage and management, which influence the system’s performance and response time.
The Role of Data Models
Different types of data models find varied applications across industries such as healthcare, retail, and finance. Conceptual models, for instance, can assist in mapping patient data in healthcare, while physical data models guide the creation of databases for patient records. In the retail industry, logical models might be utilized to structure customer and inventory data, driving AI-powered sales predictions. Meanwhile, in finance, physical models become indispensable in developing databases for complex financial transactions. These real-life applications further underscore the extensive relevance of data modeling across diverse sectors.
Data Modeling Best Practices
Despite the criticality of data modeling, certain pitfalls can lead to suboptimal AI performance. A common misstep is overlooking the need for regular model updates. As data continues to evolve, so should your models. Neglecting to incorporate changes in data trends and patterns can result in outdated and ineffective models. Regular data model reviews help keep them current and relevant.
Another frequent oversight is neglecting data security and privacy considerations while creating models. Given the sensitive nature of data handled by AI systems, robust security measures must be integral to data modeling. Prioritizing data security and privacy can help avoid potential data breaches and non-compliance issues.
Merging Data Modeling and AI Success
Regardless of one’s role in an AI initiative, understanding and implementing effective data modeling techniques is fundamental to success. As we delve further into the AI era, mastering the art of data modeling will become an increasingly significant factor in driving AI excellence.
With the help of automation tools like WhereScape, organizations can enhance their data modeling processes, resulting in more streamlined, efficient, and successful AI projects.
Optimizing Enterprise Data Management Solutions with WhereScape RED
Empowering Enterprise Data Management with WhereScape RED Choosing the best data warehouse automation software can make enterprises more scalable, accurate, and competitive. WhereScape RED is one of the most empowering enterprise data management solutions available,...
Enhancing Data Interoperability: Connecting Diverse Systems with WhereScape
Understanding Data Interoperability in Modern Enterprises Modern enterprises use countless different systems to gather, store, analyze, and process data. From varying data types to unique purposes and levels of security, a single enterprise handles data in several...
Gartner Highlights the Rise of Data Warehouse Automation
Imagine a world where the manual, tedious tasks of data warehouse development are a thing of the past. This isn't a far-off fantasy but a present-day reality, thanks to advances in Data Warehouse Automation (DWA). Gartner's latest report by analyst Henry Cook,...
Experience the Power of WhereScape 3D 9.0.3: New Features and Improvements
We’re thrilled to introduce our latest iteration of WhereScape 3D! Version 9.0.3 brings a host of new features and enhancements designed to make your data warehousing journey smoother, faster, and more efficient. Let’s dive into the details of what you can expect from...
Ahead of the Curve: Future Trends in Data Automation and WhereScape’s Pioneering Solutions
The Evolving Landscape of Data Automation As new technologies emerge and existing tools constantly change and improve, the world of data automation transforms rapidly. Even the most well-versed data teams find themselves disoriented and overwhelmed in the face of...
Investing in Data Automation: A Strategic Approach to Business Growth
Unlocking Growth: The Strategic Advantage of Data Automation Organizations reaping the benefits of data automation stay ahead of industry trends and improve the efficiency of their operations and decision-making. Data automation tools offer a strategic advantage for...
Data + AI Summit 2024: Key Takeaways and Innovations
The Data + AI Summit 2024, hosted by Databricks at the bustling Moscone Center in San Francisco, has concluded with remarkable revelations and forward-looking innovations. Drawing over 16,000 attendees in person and virtually connecting over 60,000 participants from...
WhereScape RED 10.1 is Here: Enhanced Scheduling and Customization
We’re proud to announce the highly anticipated WhereScape RED 10.1 is now available, and it’s packed with exciting new features and enhancements designed to make your data warehousing experience more efficient and enjoyable. Let's take a closer look at what’s new and...
Supercharging Data Integration: The WhereScape and Databricks Advantage
The demand for robust data management systems has never been higher, and Databricks has quickly become a favored choice for cloud-based solutions. Its powerful capabilities make it a top contender for managing large-scale data, but when combined with WhereScape's...
Empowering Customer Success: WhereScape’s Comprehensive Support and Training Resources
Enhancing Operational Success with WhereScape’s Support Systems At WhereScape, we understand that a data warehouse is only useful to the extent that it is understood. In order to drive your organization closer to your key goals and objectives, you need full mastery of...
Related Content
Optimizing Enterprise Data Management Solutions with WhereScape RED
Empowering Enterprise Data Management with WhereScape RED Choosing the best data warehouse automation software can make enterprises more scalable, accurate, and competitive. WhereScape RED is one of the most empowering enterprise data management solutions available,...
Enhancing Data Interoperability: Connecting Diverse Systems with WhereScape
Understanding Data Interoperability in Modern Enterprises Modern enterprises use countless different systems to gather, store, analyze, and process data. From varying data types to unique purposes and levels of security, a single enterprise handles data in several...
Gartner Highlights the Rise of Data Warehouse Automation
Imagine a world where the manual, tedious tasks of data warehouse development are a thing of the past. This isn't a far-off fantasy but a present-day reality, thanks to advances in Data Warehouse Automation (DWA). Gartner's latest report by analyst Henry Cook,...
Experience the Power of WhereScape 3D 9.0.3: New Features and Improvements
We’re thrilled to introduce our latest iteration of WhereScape 3D! Version 9.0.3 brings a host of new features and enhancements designed to make your data warehousing journey smoother, faster, and more efficient. Let’s dive into the details of what you can expect from...